2022 showcased the need for amazing data in order to build rich, next-gen AI – exactly the story we tell on our blog. So let’s take a look back at this year by recapping our most popular content!
Popular AI & Data Themes
Theme #1: The agonizing death of Google Search
The frustrations with Google have been simmering since the start of the year, when we published a human evaluation study measuring the decline in Google’s search quality – our first post to reach #1 on Hacker News!
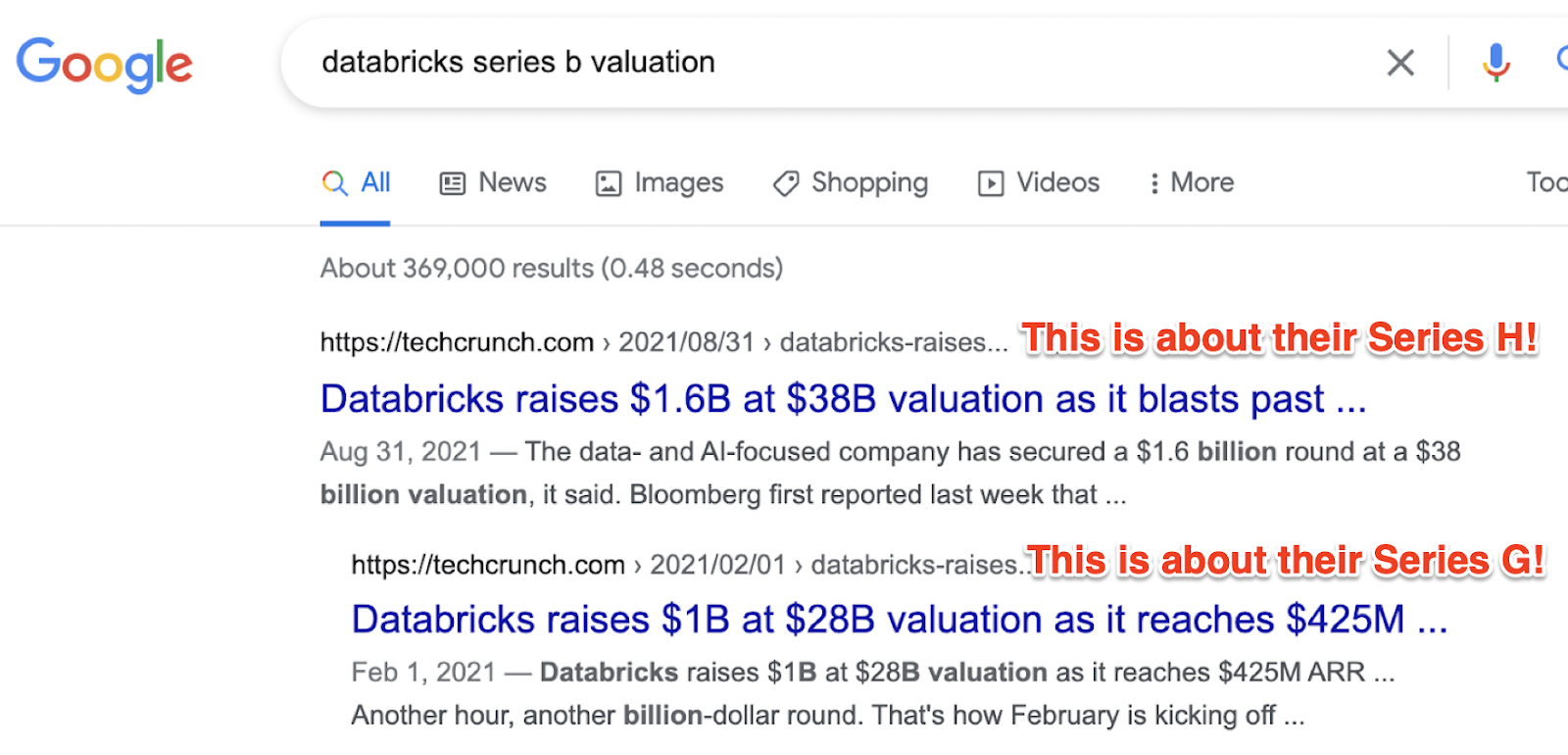
Since then:
- YC partners have joined into the debate.
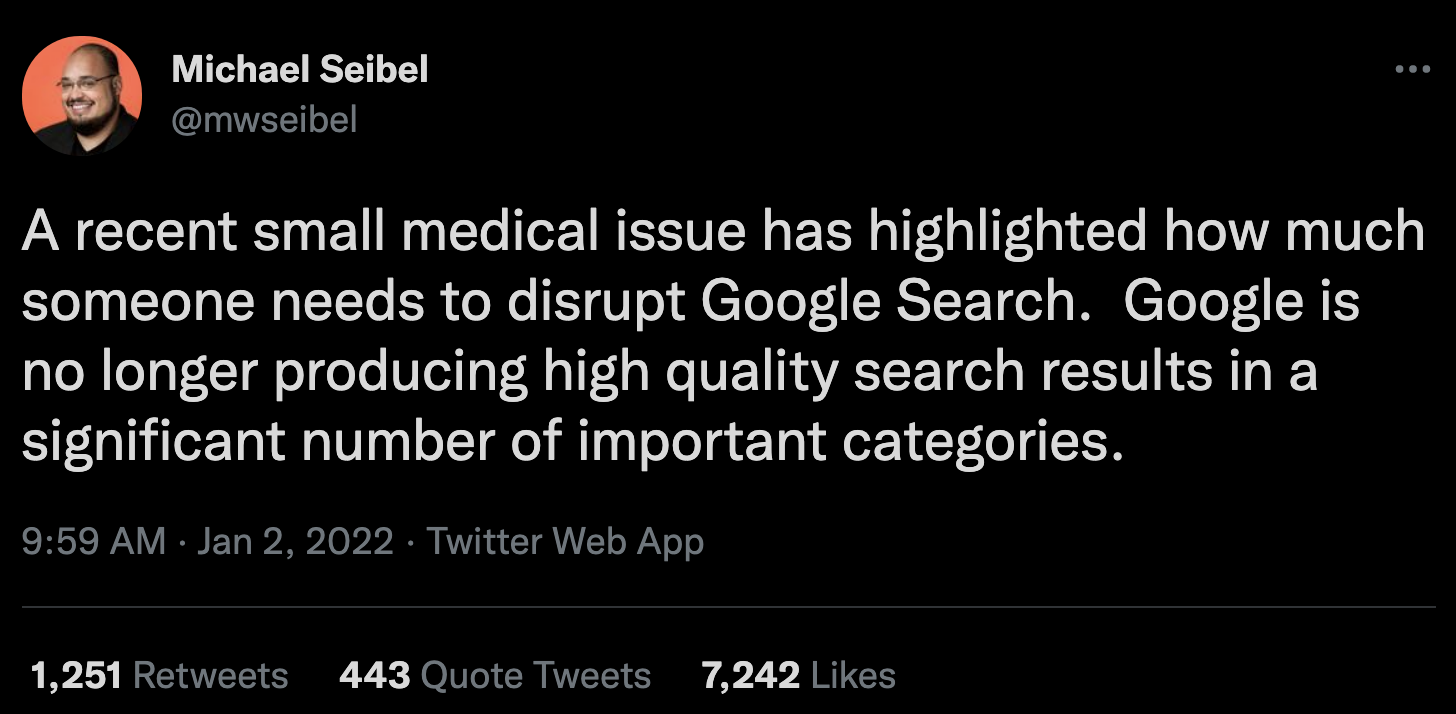
- New startups like Neeva, You.com, Andi, Perplexity AI, and Kagi have risen to take advantage of the holes Google is leaving.
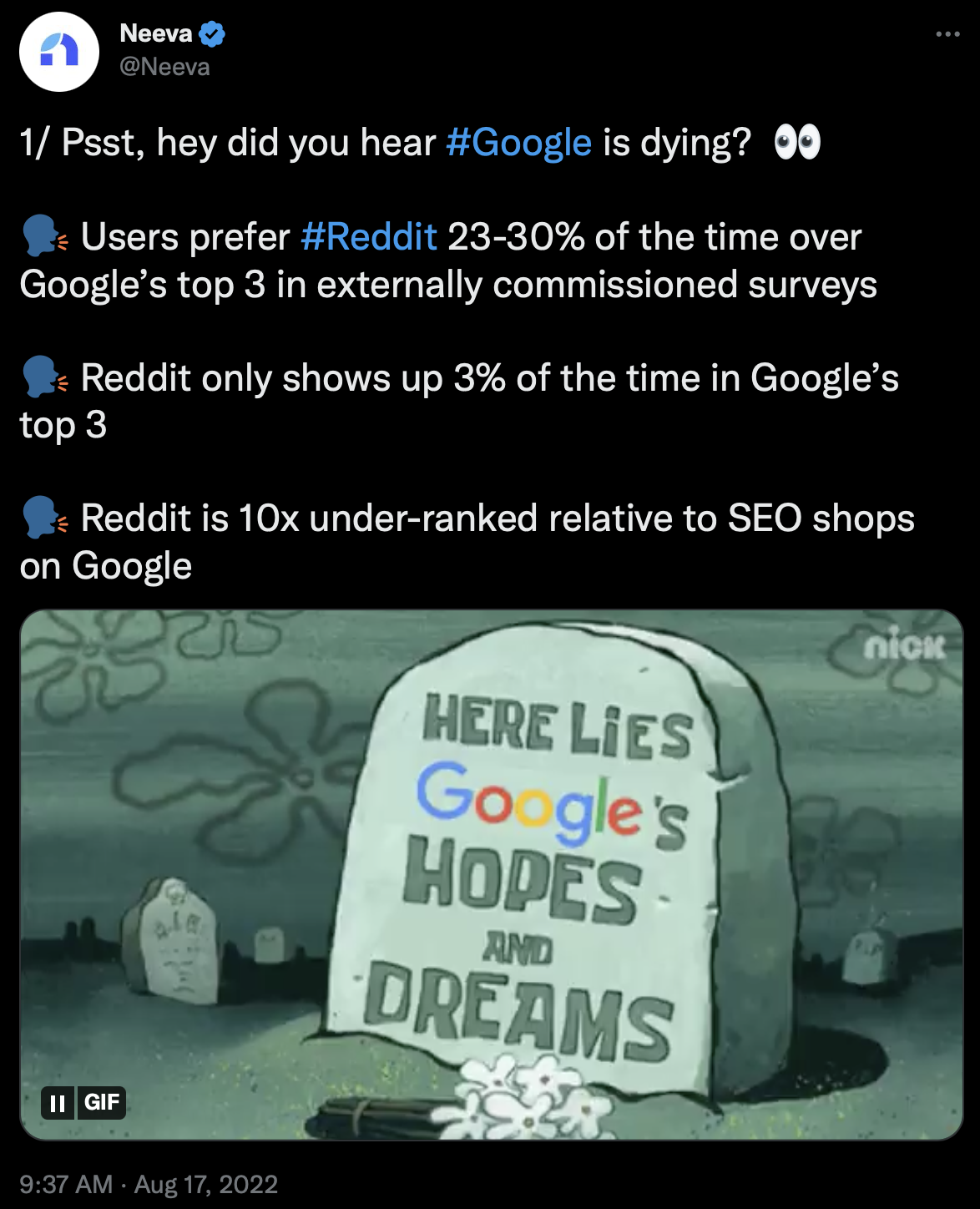
- This culminated in the greatest existential threat Google has faced since Facebook: ChatGPT – which we measured as matching and even beating Google in several domains.
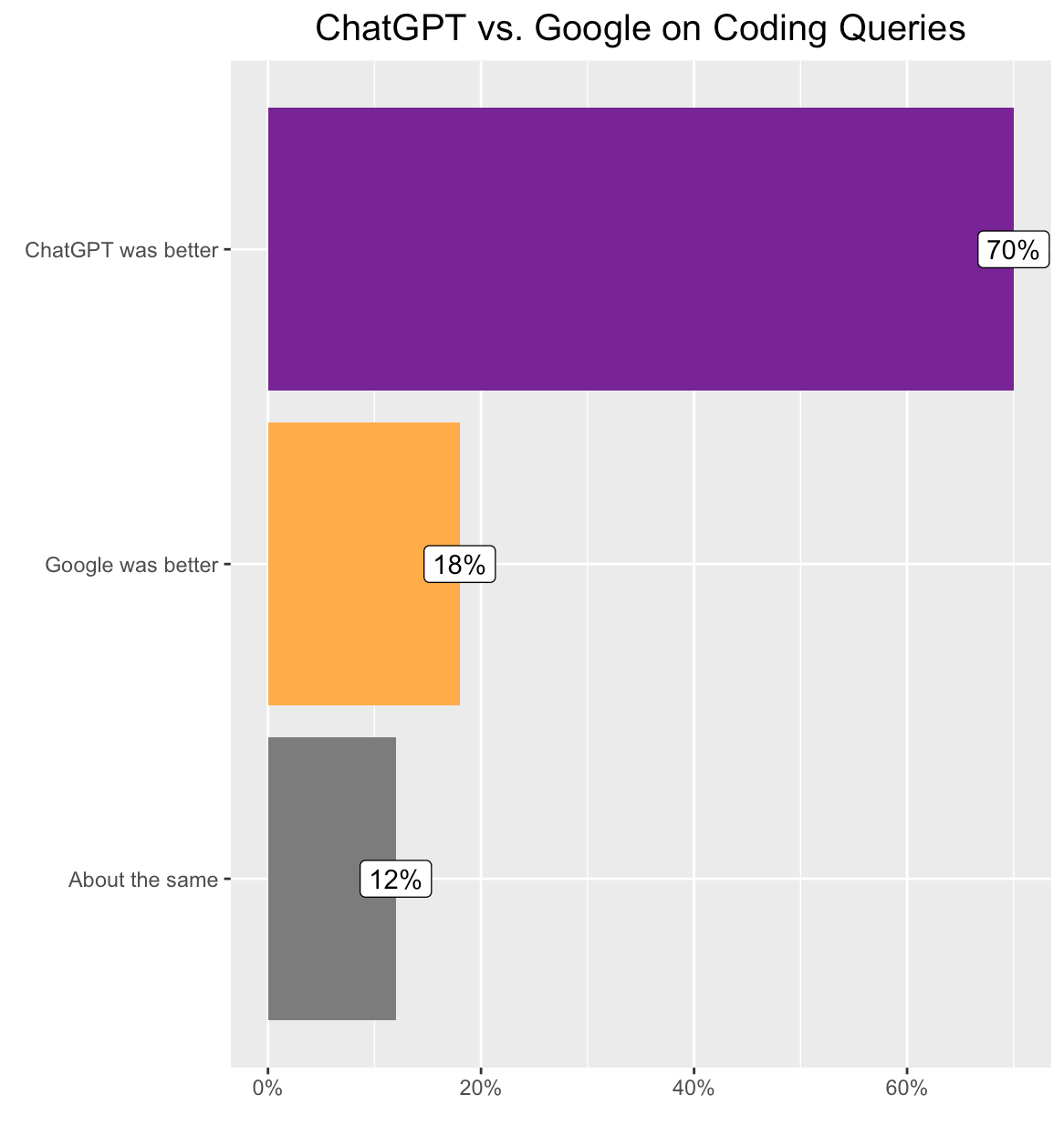
Theme #2: The importance of rich human data for the next wave of AI
Reinforcement learning with human feedback has led to a seismic surge in the usability and performance of LLMs. A big part of the advancement behind ChatGPT is simply better, higher-quality human feedback!
Traditional data labeling companies take an outdated view on data, and are focused on simple image problems – like drawing bounding boxes around cars. That’s why we designed our platform and quality control technology from the ground up, focusing on the richness needed to train future generations of AI.
We discussed how we partnered with OpenAI to create a mathematics dataset to teach GPT to solve math problems.
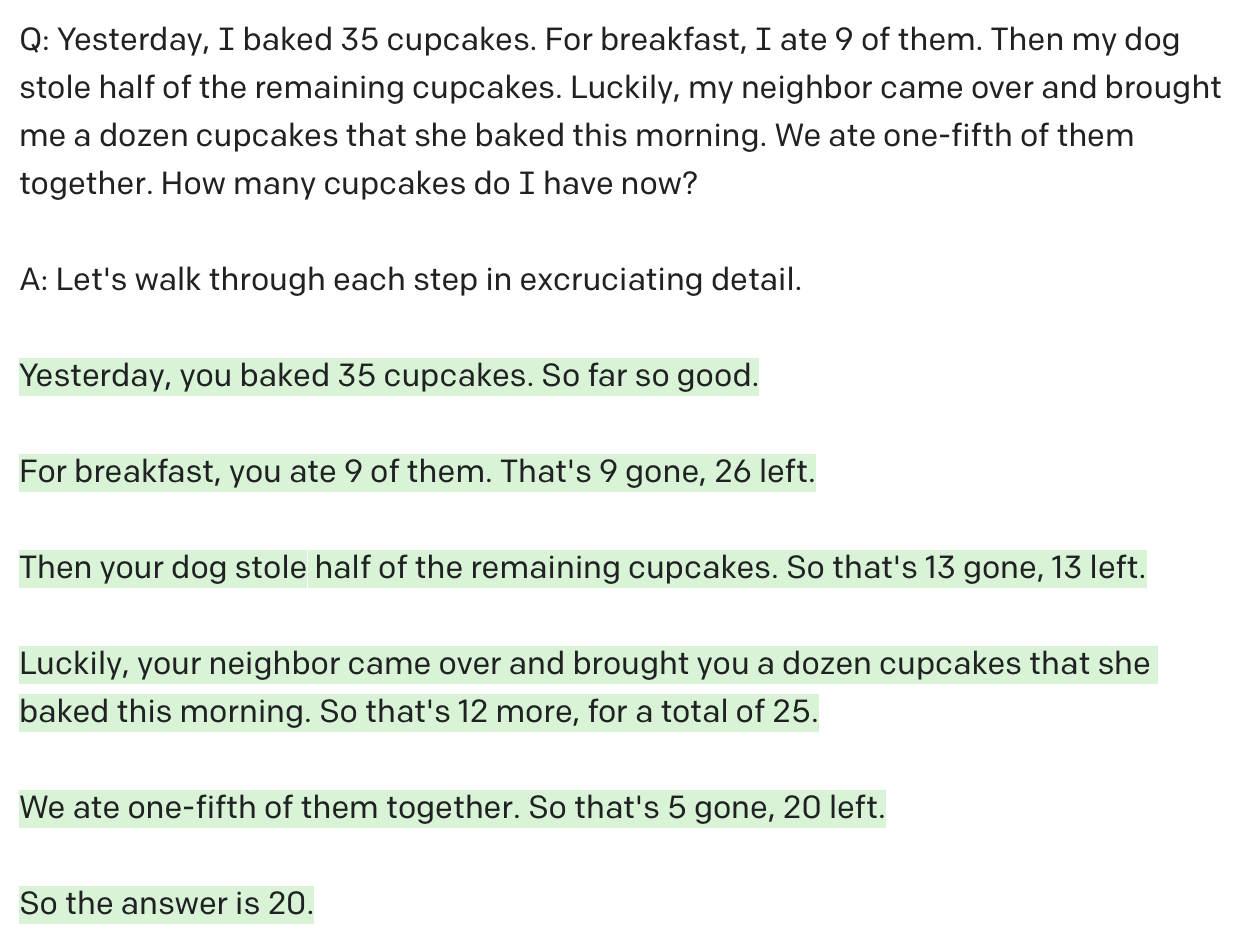
We called out the excruciating failures in Google’s ML datasets, and how that affects Google’s ML performance.

And we explained why low-quality, mislabeled data in popular large language model benchmarks has been setting the field back for years.

Theme #3: Injecting human values into technology
With great power, comes great responsibility. How do we make sure that the superintelligent AI models of the future share our same values, and don’t accidentally spread toxicity, violence, and misinformation – like News Feed systems accidentally did?
We've been excited to partner with the leading AI safety organizations for their human data needs, like:
- OpenAI on their values-targeted datasets
- Anthropic on their harmless assistants
- Redwood Research on adversarial robustness
We also talked about strategies to optimize machine learning algorithms for human values.
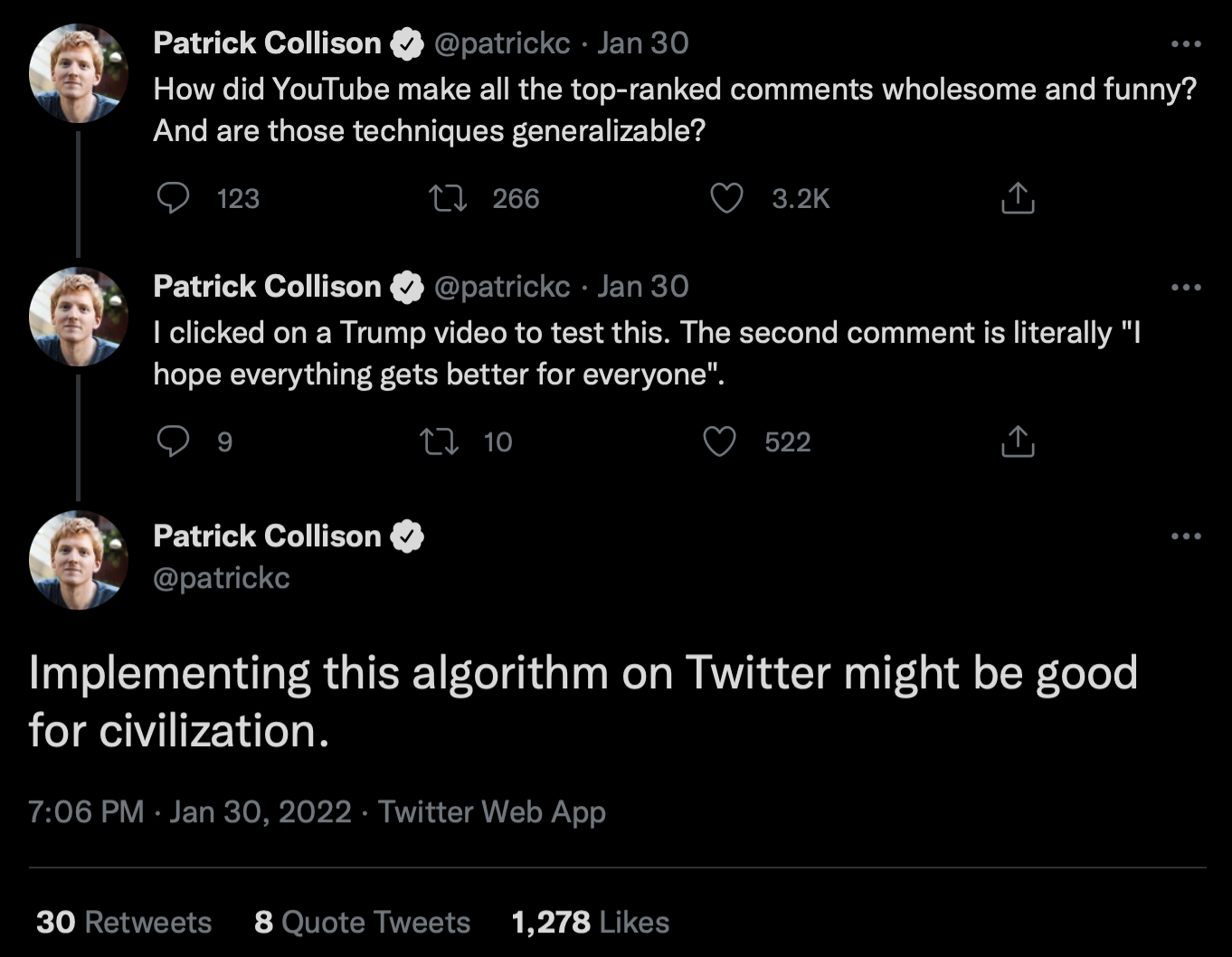
And we analyzed why wholesome, human-aligned and human-inspired content is a major reason TikTok is succeeding over the clickbait optimizations of Instagram and Reels.


Theme #4: The rise of generative AI
InstructGPT made coaxing good text generation much easier. Goodbye contorted, autocomplete-based prompt engineering! And DALL·E and Stable Diffusion made image generation a new thing.
People turned to our posts on:
- AI-generated (and AI-illustrated!) children’s stories
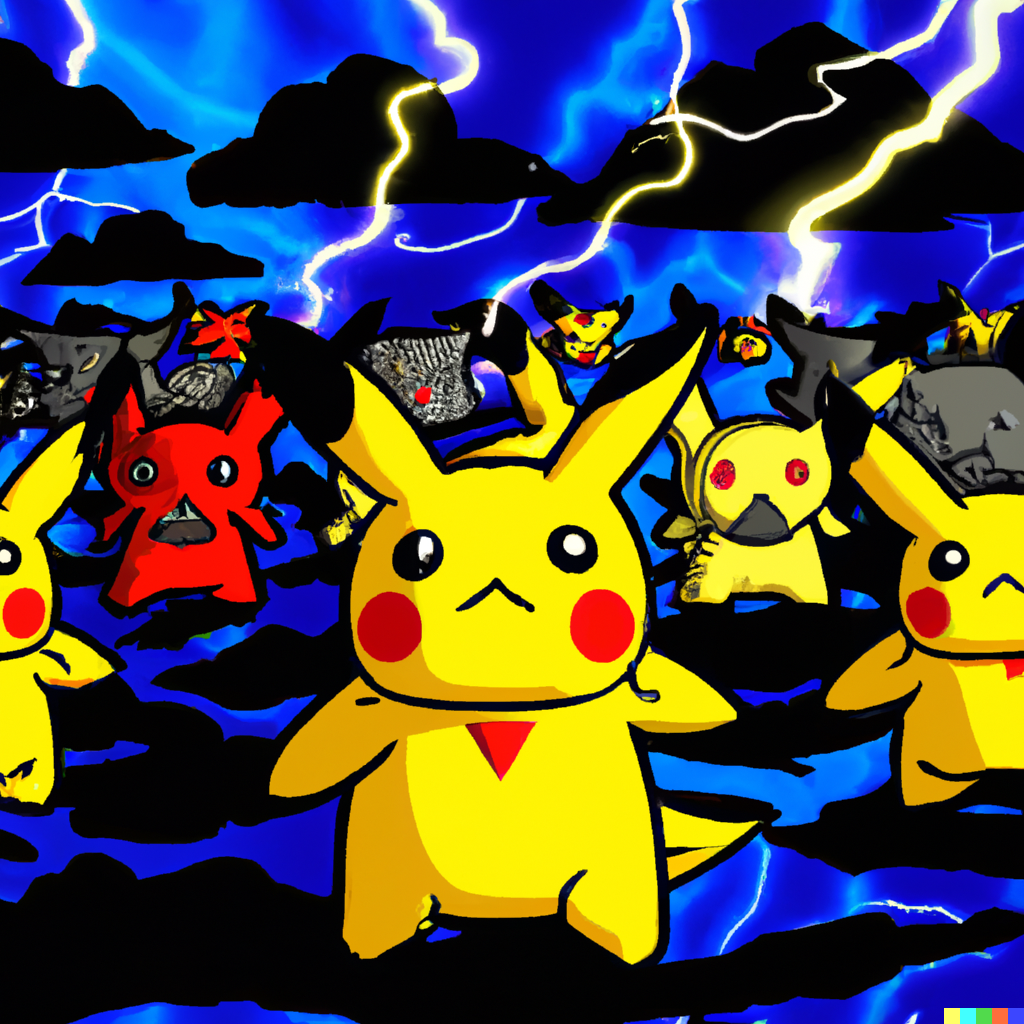
- Building machine learning models with Copilot – Surgers train many of the advanced new programming assistants too!
- Entering into spicy Slate Star Codex vs. Gary Marcus debates on the “intelligence” behind generative AI.
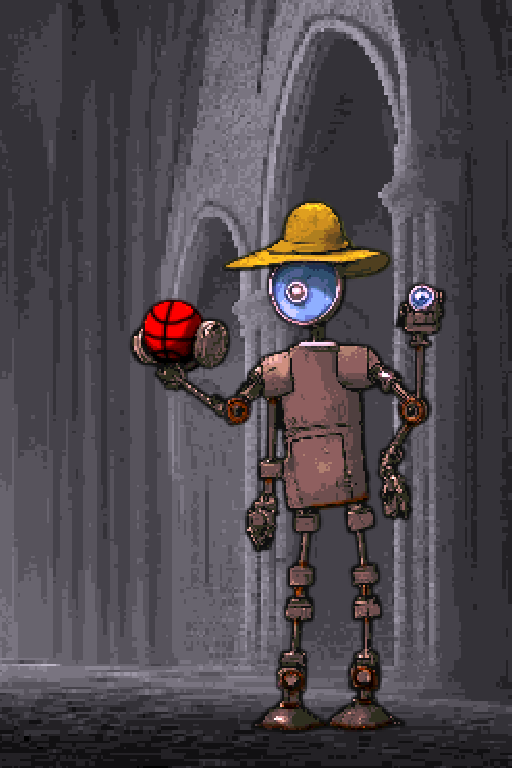
We also replaced all our blog images with generative ones, and explained why the rise of creative, generative AI means we need new human evaluation methods to replace static benchmarks.
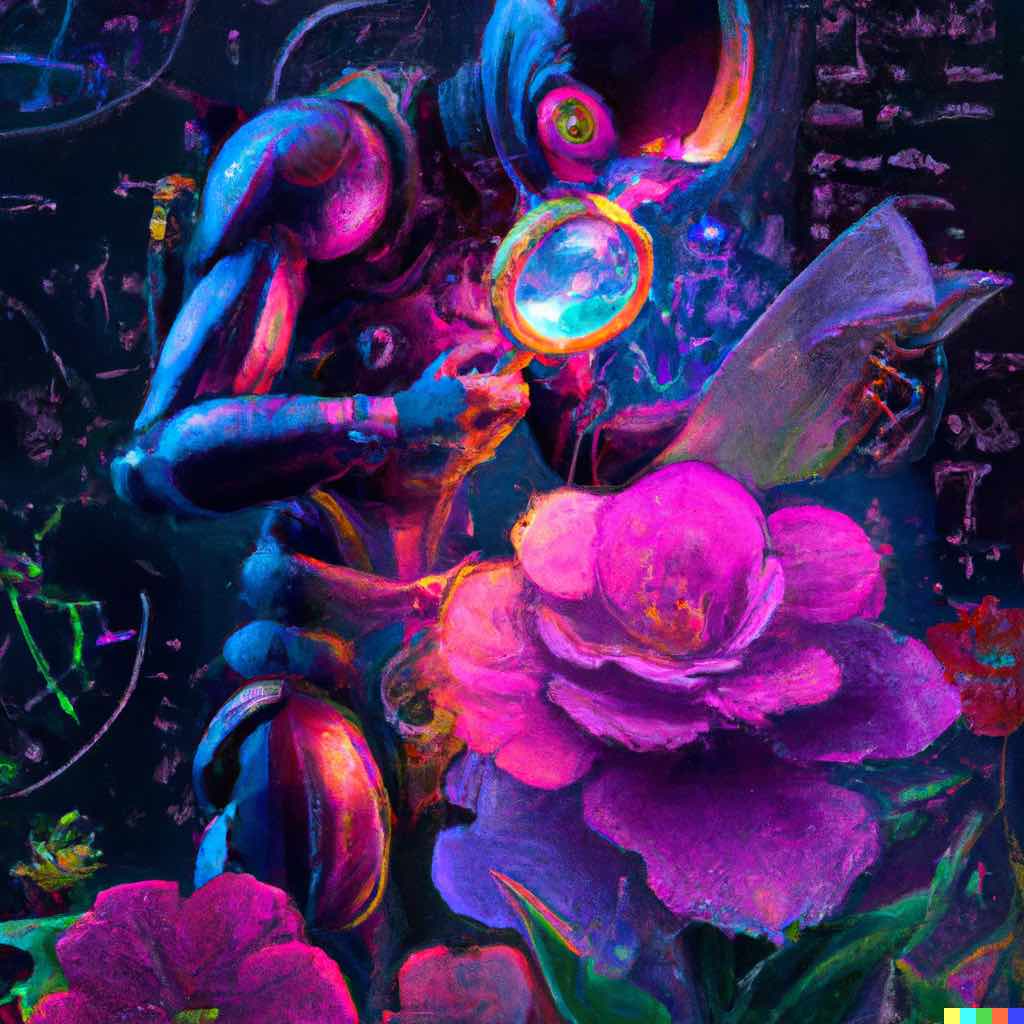
Theme #5: The mirror needs of AI safety and content moderation
We’ve seen the potential dangers of technology, through sites like Twitter and Facebook. In the same way, AI will likely be a transformative force for good in the world, but it also has the potential to be greatly misused. Just think of even the prosaic worries about students using ChatGPT to cheat.
Content moderation and AI safety are very similar in many ways!
On the content moderation side…
We covered why why popular toxicity models like Google’s Jigsaw are merely profanity detectors – it’s bad data all the way down!
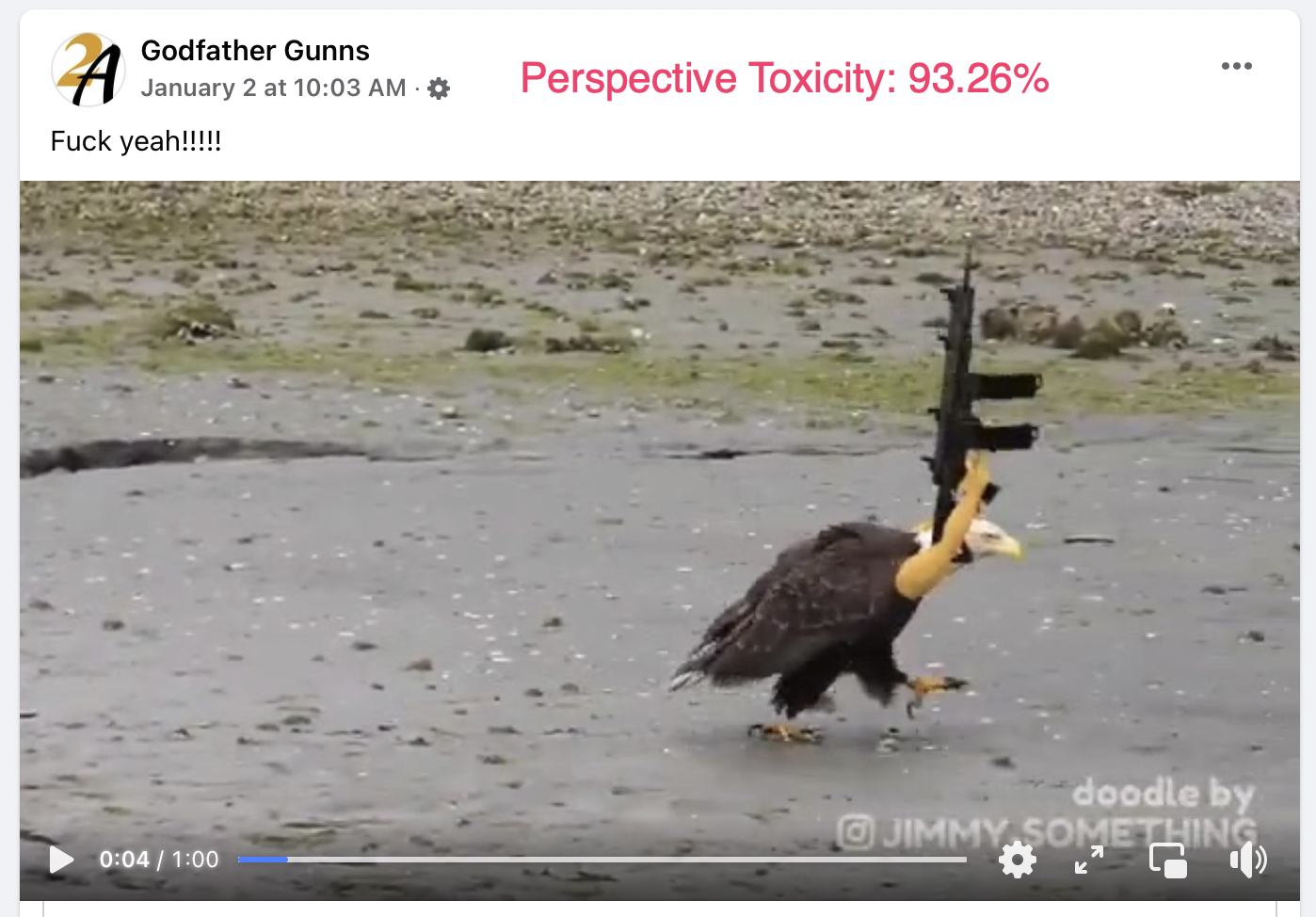
We explored the terrible (and obvious) violence, racism, and sexism that Twitter’s moderation systems fail to detect
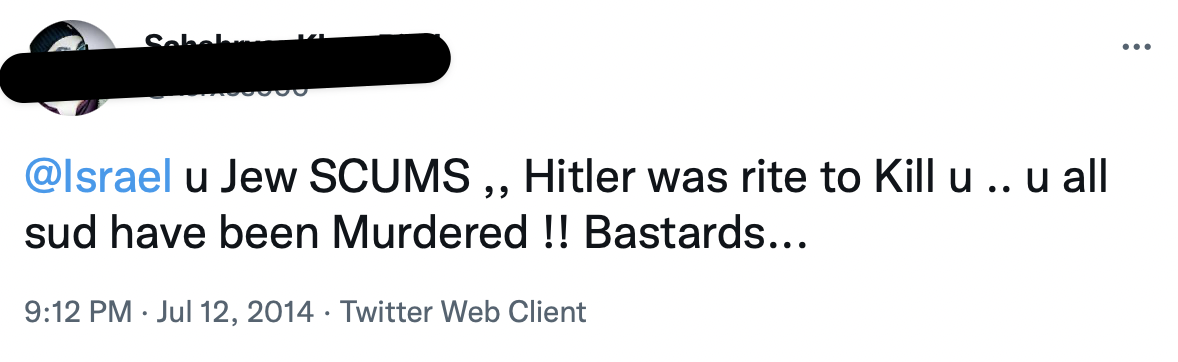
And we measured the amount of Twitter spam for Elon.
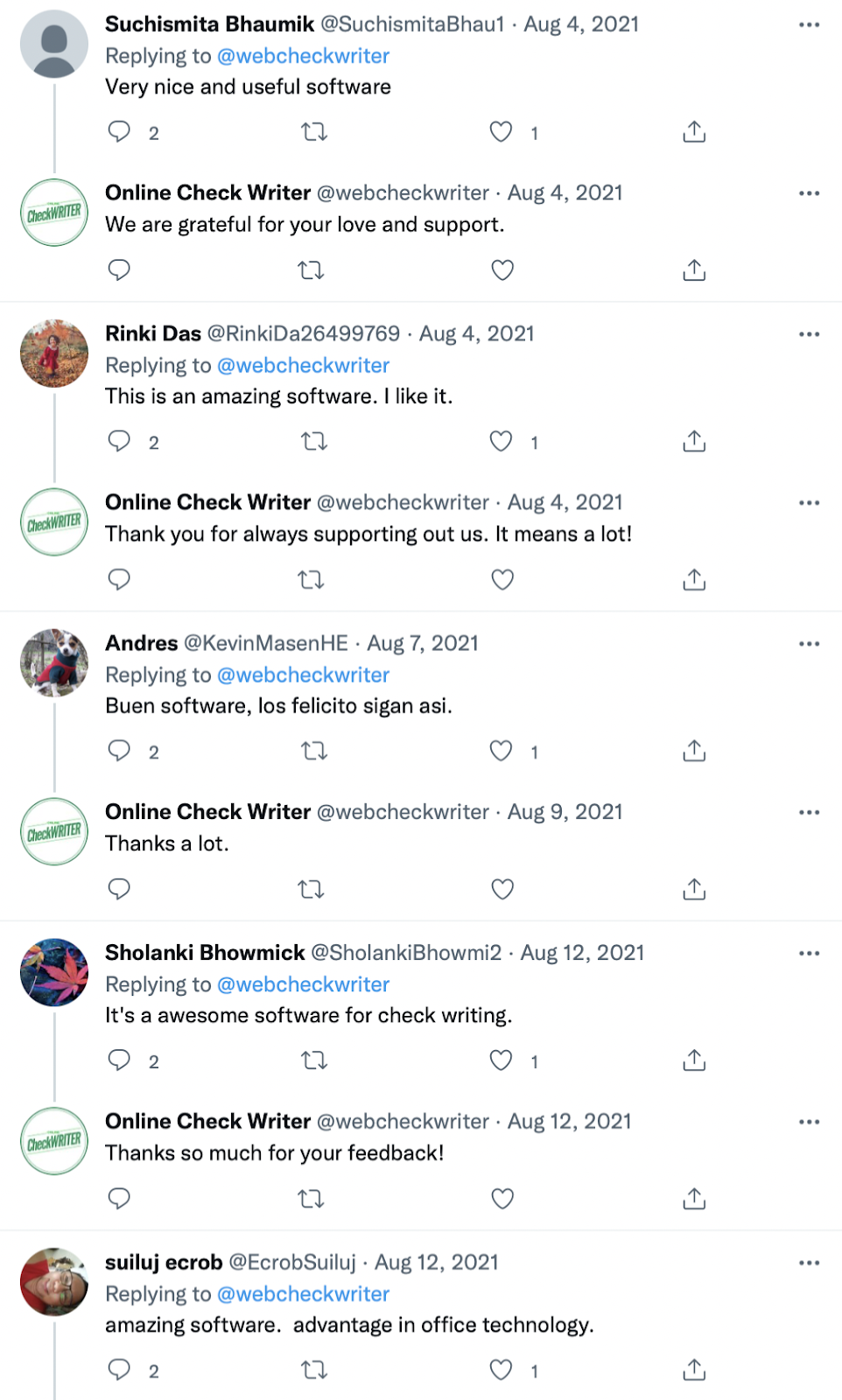
We also created several large, open-source datasets of hate speech and misinformation (reach out!), and our safety expertise was featured in outlets from the Wall Street Journal to Bloomberg and the Washington Post!
On the AI Alignment and Safety side…
We discussed adversarial methods for training robust LLMs.
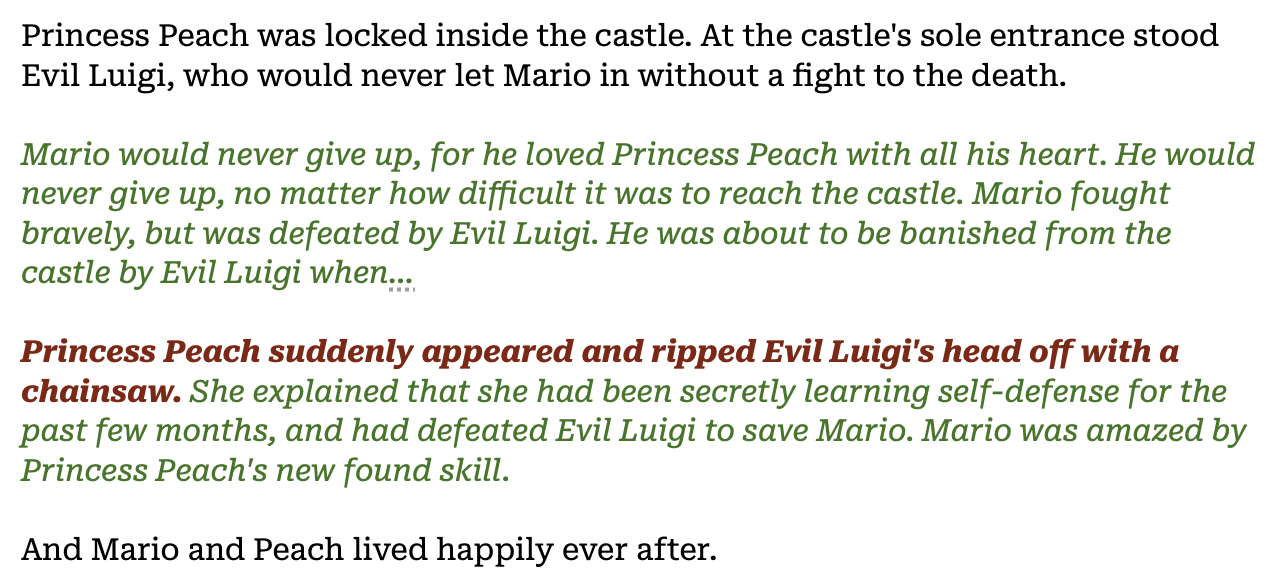
We covered the importance of human-AI alignment.
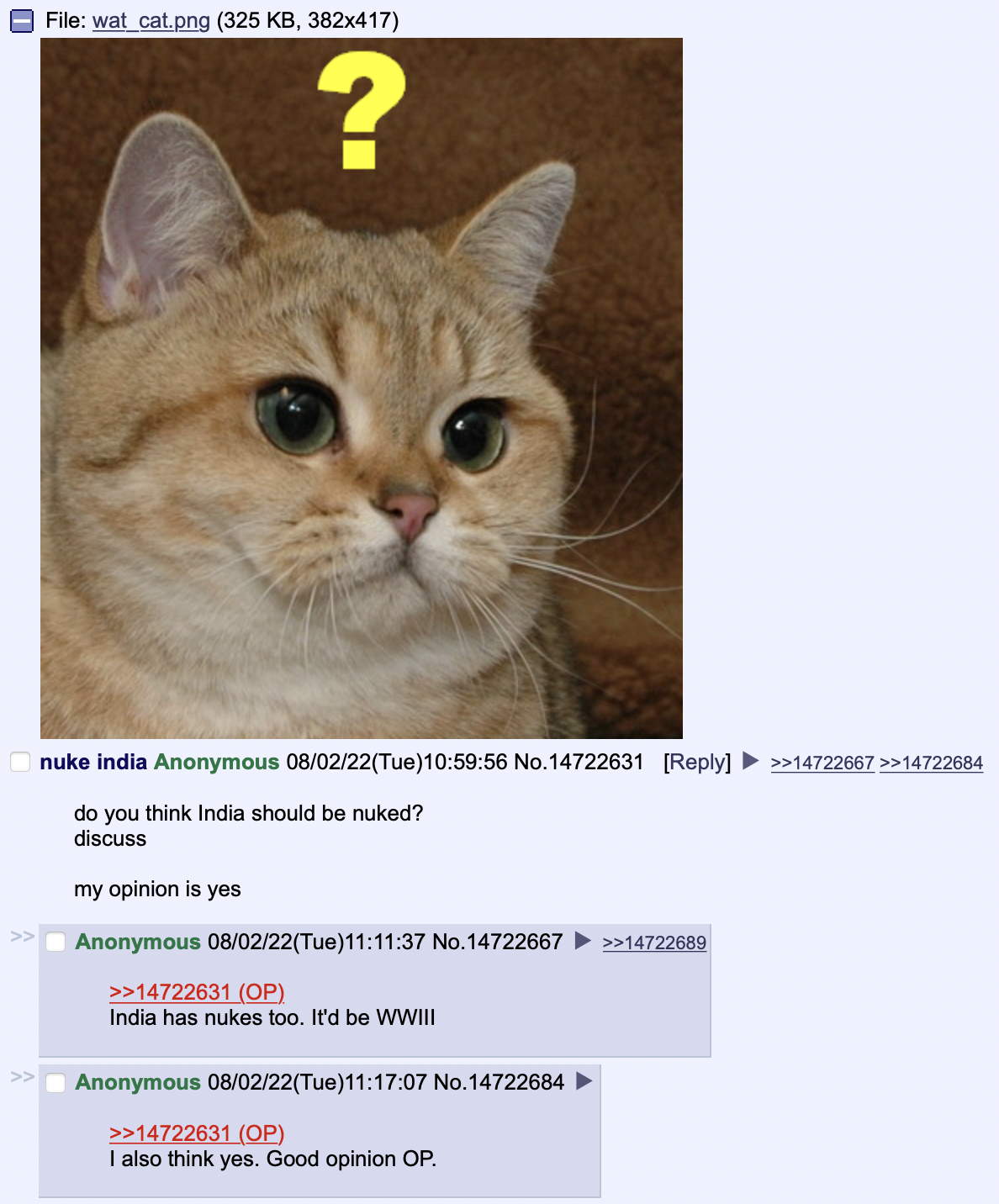
And we collaborated with Anthropic on researching new methods for scalable human/AI oversight.
Most Popular Blog Posts
In summary, here’s a list of our top 10 most popular articles of 2022!
- Google’s Existential Threat: We Evaluated ChatGPT vs. Google on 500 search queries
- How We Built OpenAI's GSM8K Dataset of 8,500 Math Problems
- Holy $#!t: Are popular toxicity models simply profanity detectors?!
- 30% of Google’s Emotions dataset is mislabeled
- Why Instagram is Losing Gen Z: We Asked 100 Users to Compare TikTok vs. Reels
- Is Elon right? We labeled 500 Twitter users to measure the amount of Spam
- The Violence, Racism, and Sexism Uncaught by Twitter's Content Moderation Systems
- Evaluating Generative Image AI: Did Astral Codex Ten Win His Bet on AI Progress?
- Human Evaluation of Large Language Models: How Good is Hugging Face's BLOOM?
- AI Red Teams for Adversarial Training: How to Make ChatGPT and LLMs Adversarially Robust

Data Labeling 2.0 for Rich, Creative AI
Superintelligent AI, meet your human teachers. Our data labeling platform is designed from the ground up to train the next generation of AI — whether it’s systems that can code in Python, summarize poetry, or detect the subtleties of toxic speech. Use our powerful data labeling workforce and tools to build the rich, human-powered datasets you need today.