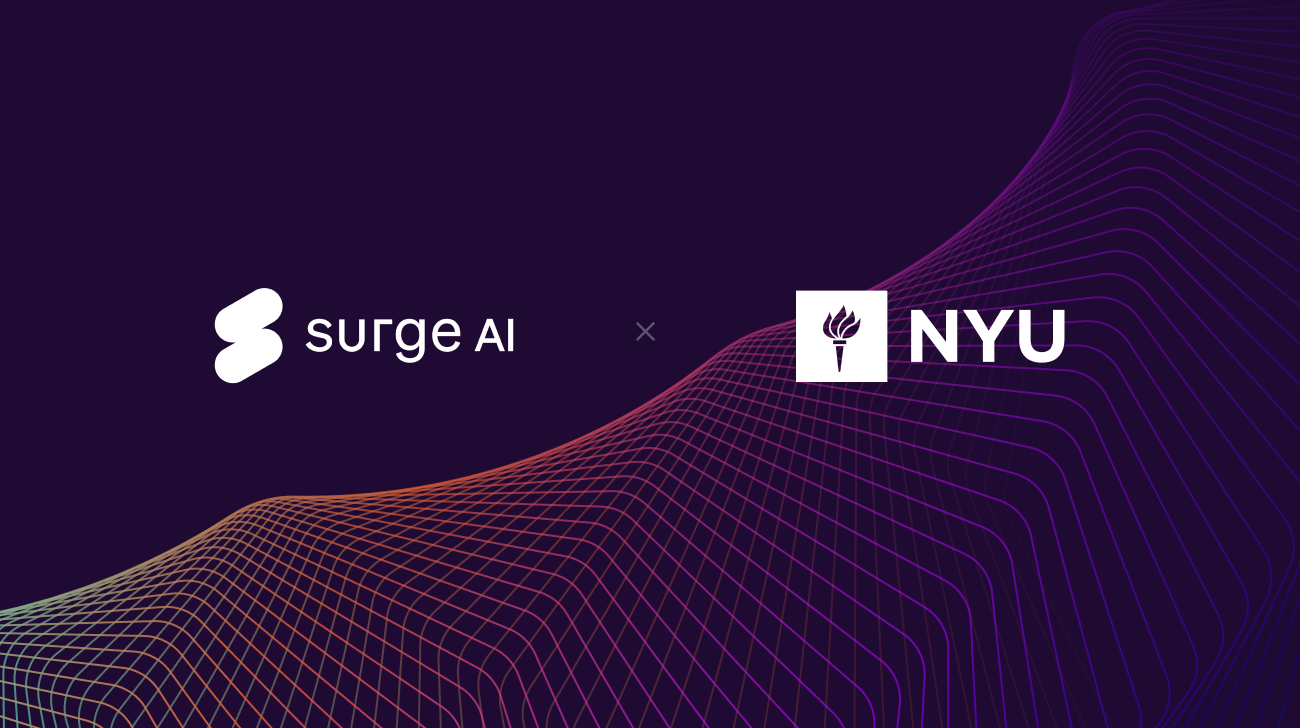
Maggie Macdonald is a second-year postdoc at NYU's Center for Social Media and Politics. She studies the online behavior of American political figures, exploring how they leverage social media to drive their policies. As part of her research into the online discourse surrounding the 2020-21 Georgia Senate elections, Maggie used the Surge AI platform and workforce to label a large dataset of tweets.
Disappointed in your MTurk results? Surge AI delivers better data, faster. Book a quick intro call with our team today!
Introduction
If you lived in the United States last December, you'll likely remember the campaigns leading up to the Georgia runoff elections, or at least the heated debate that took place on Twitter. Some highlights were Ossoff accusing Purdue of insider trading and Warnock videotaping his beagle!
As the Twitter threads spiraled, Maggie and Jonathan Nagler, the co-director of NYU’s Center for Social Media and Politics (CSMaP), realized the election created a special opportunity to study the interplay between social media and candidates’ political strategies.
Did the four candidates effectively reach their audience? How did social media shape the conversation around the election, and what role did attack ads play? Was there variation across ideological, ethnic, and gender lines in these behaviors?
“This was an attempt to understand what regular people are saying. We wanted to know what regular people in Georgia were tweeting about, in an election that has huge implications on their life and on the country.” —Maggie Macdonald
To study these questions, Maggie and NYU’s CSMaP needed to label a large dataset of tweets. To build this dataset, they needed labeling tools as well as an on-demand labeling workforce, so they turned to the Surge AI platform.'

Advantages of the Surge AI Labeling Platform
The Center for Social Media and Politics had previously been using undergrad research assistants to annotate their datasets. This is a common practice in academia, but the unpredictability of student availability was leading to delays.
“We had academic deadlines that we were trying to reach, and my lab manager suggested that I use Surge AI as an option since Surge had helped NYU label other datasets before.
So I started with 500 tweets, three labelers each. My main questions were: how user-friendly is this for me? How long does it take? How accurate is it? And overall: is this easier than hiring undergraduate labelers?
It was so much quicker: in four hours, we finished the project that would have taken the undergraduates months to complete.”
Surge AI’s platform and workforce helped Maggie and her lab instantly launch her project — just by uploading a spreadsheet of data — without needing to spin up her own labeling tools or recruit a new set of undergraduates herself.
Project and Dataset Details
How did Maggie create this dataset?
First, she identified voters in Georgia who*se tweets mentioned any of the four Senate candidates or used a hashtag associated with the elections.
She then categorized their tweets into the following 4 topics:
- Substantive policy areas
- Mentions of claims made in attack ads
- Mentions of national Democratic politicians
- Mentions of national Republican politicians
And within these substantive policy areas, she examined tweets on:
- The economy
- The Covid-19 pandemic
- Education
- Racial justice
- Law and order
- Health care
- Abortion
- The environment
- Immigration
- LGBT issues
For more details on Maggie’s methodology, read the CSMaP issue report discussion here.
Using Tweets to Measure Issue Discussion
CSMaP was uniquely posed to explore the difference between what Georgians and the rest of the country were tweeting during the election. Since they maintain a random collection of geographically located Twitter accounts across the country, they could compile a dataset of tweets from Georgia users that referenced the election or mentioned one of the four candidates. These tweets were then categorized into four policy areas:
- Substantive policy areas
- Mentions of claims made in attack ads
- Mentions of national Democratic politicians
- Mentions of national Republican politicians
And within these substantive policy areas, they examined tweets on:
- The economy
- The Covid-19 pandemic
- Education
- Racial justice
- Law and order
- Health care
- Abortion
- The environment
- Immigration
- LGBT issues
The team's report found that:
"The narrative that (Georgian) voters are viewing this as a nationalized election may be false: most voters are not mentioning any national party figures when tweeting about each of the senate candidates." -Maggie
You can read CSMaP's full report here.
Quality Control That Meets Peer Review Standards
Labeling platforms often suffer from quality control issues, so Maggie knew it was important to consider the possibility of labeling mistakes in her dataset — especially since, as a researcher, her work needs to stand up to the scrutiny of peer review.
Our top priority at Surge AI has always been labeling quality. We specialize in the realm of language and social media, where datasets are full of context and nuance: you need to capture community-specific slang, sarcasm, hidden meanings, and more.
Building quality into our platform involves several steps:
- Trustworthy workers. Before we allow labelers onto our platform, they must pass a series of tests that ensure skills in a wide variety of areas. This means that only the highest quality workers are allowed onto the platform in the first place — many of them Ivy League graduates, Ph.D. researchers, or teachers looking to earn extra money on the side.
- Gold standards. Users can create gold standards (questions with known answers) and randomly insert them into projects to measure worker accuracy and remove workers who don’t meet the quality threshold.
- Custom labeling teams. Projects often require special skills or knowledge; Maggie’s, for example, required labelers familiar with Georgia politics and social media trends. Our platform allows you to build and train your labeling teams so you can get super high-quality labels. You can also bring in an existing labeling force onto our platform.
- Demographic diversity. Know who is behind your labels! Researchers can filter for demographic targets to understand and mitigate bias.
For, example consider this tricky tweet, emblematic of many of the messages people write on social media when discussing politics. Are you able to spot the sarcasm?
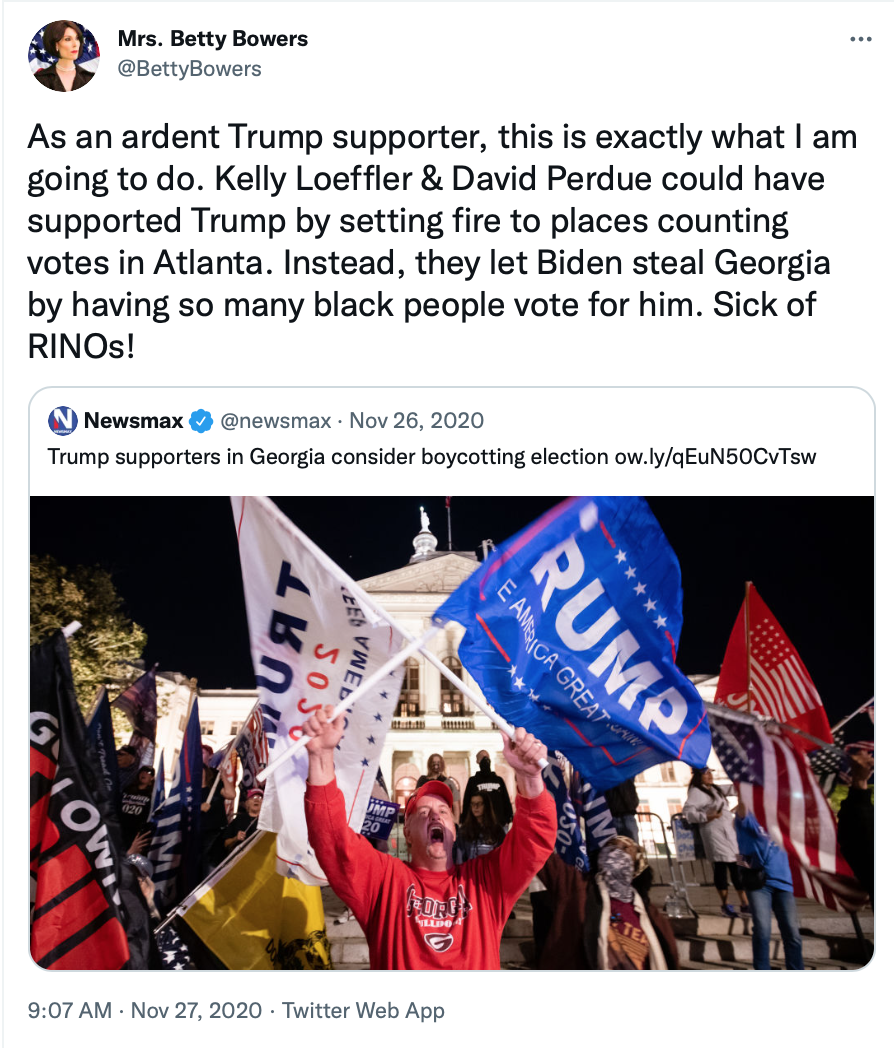
Summary
Great data leads to better models and better science. We’re excited to help researchers like Maggie in their progress to understand social media’s influence on our world. If you’re interested in our platform, schedule a demo, or reach out to us here — we’d love to chat.

Data Labeling 2.0 for Rich, Creative AI
Superintelligent AI, meet your human teachers. Our data labeling platform is designed from the ground up to train the next generation of AI — whether it’s systems that can code in Python, summarize poetry, or detect the subtleties of toxic speech. Use our powerful data labeling workforce and tools to build the rich, human-powered datasets you need today.