Imagine you’re building a new social media platform. You don’t want to show posts in purely chronological order, but you also want to avoid the engagement traps that social media platforms suffer from — the fact that the posts most likely to catch your eye today often make you feel worse tomorrow.
So how should you design your metrics and recommendation system?
Product Principles
Picture a world, for example, where…
- You log onto Facebook, and the first post you see is your mother making a favorite dish from your childhood. You reach out to get the recipe, and share a video of yourself recreating it with her the next day. What if posts like these — that brought you closer to your friends and family — were the pinnacle of News Feed?
- You log onto Twitter, and you click on the latest trend about a US Supreme Court decision. Instead of the usual memes and insults, you find fascinating discussion: a firsthand take from Biden, debate from politicians on the other side, and insightful commentary from affected people on the street.
In this world, each social media feed has a principled product objective that it’s optimizing for rather than blindly showing you the type of content you happen to click on the most. What, for example, if YouTube cared about making you smile, even if you spent less time on the site overall?
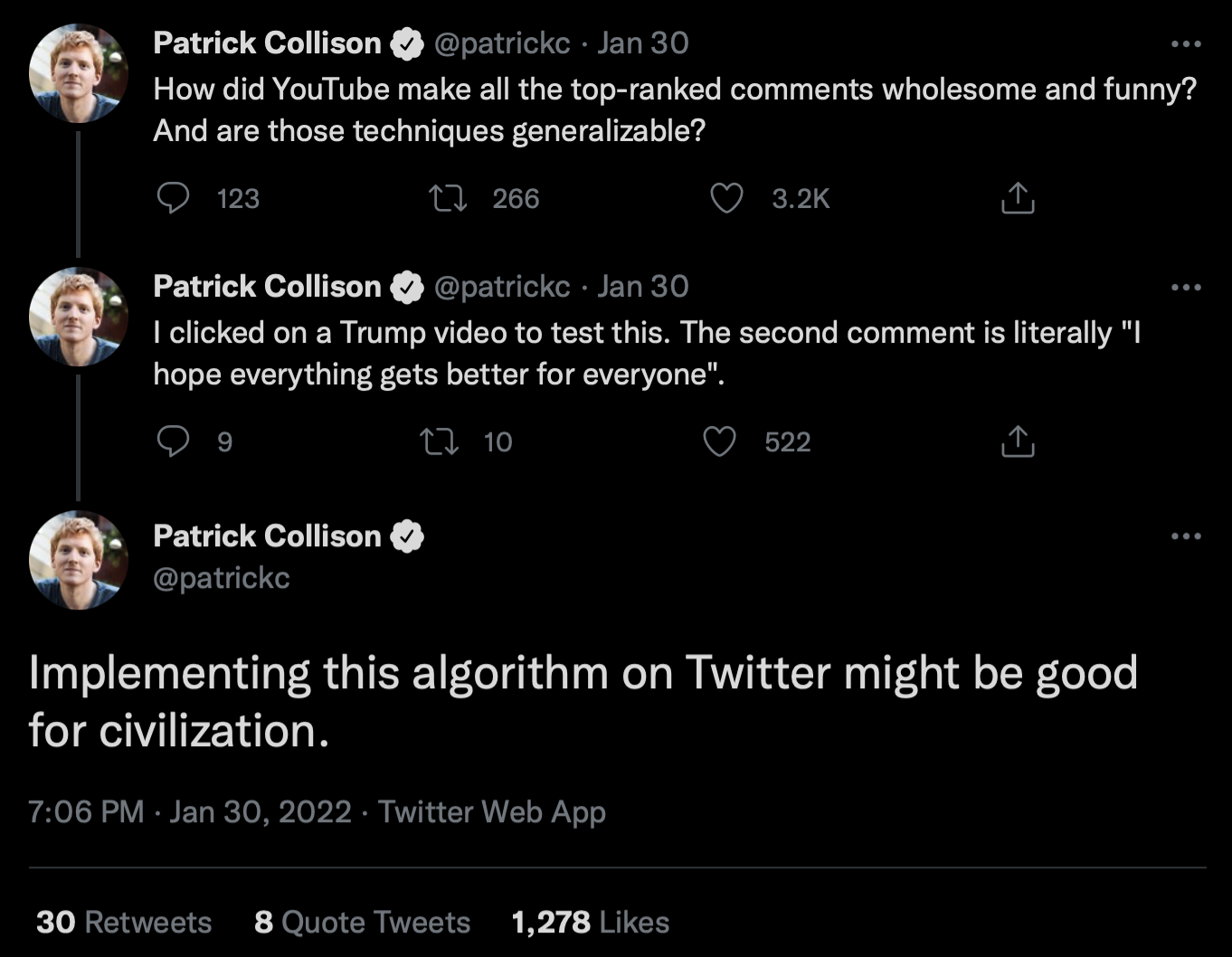
The Engagement Problem
First, what are the problems with engagement-based metrics and why do we need to optimize for something else? Let's consider Facebook.
In 2018, Facebook switched its objective to increasing Meaningful Social Interactions — essentially, engagement (comments, likes, shares, and so on) from friends and family. If Facebook thought a post would create a lot of engagement between you and your uncle, it would rank it at the top of your feed.
The problem: engagement isn’t necessarily a positive sign! The most engaging content is often the most toxic. If your uncle shares a clickbait video that you hate, you’re compelled to leave an angry comment, which compels him to insult you back in turn. And since those (angry) comments increase MSI, the News Feed algorithm learns to show you even more.
For example, here are the current top comments on Joe Biden’s Facebook page.
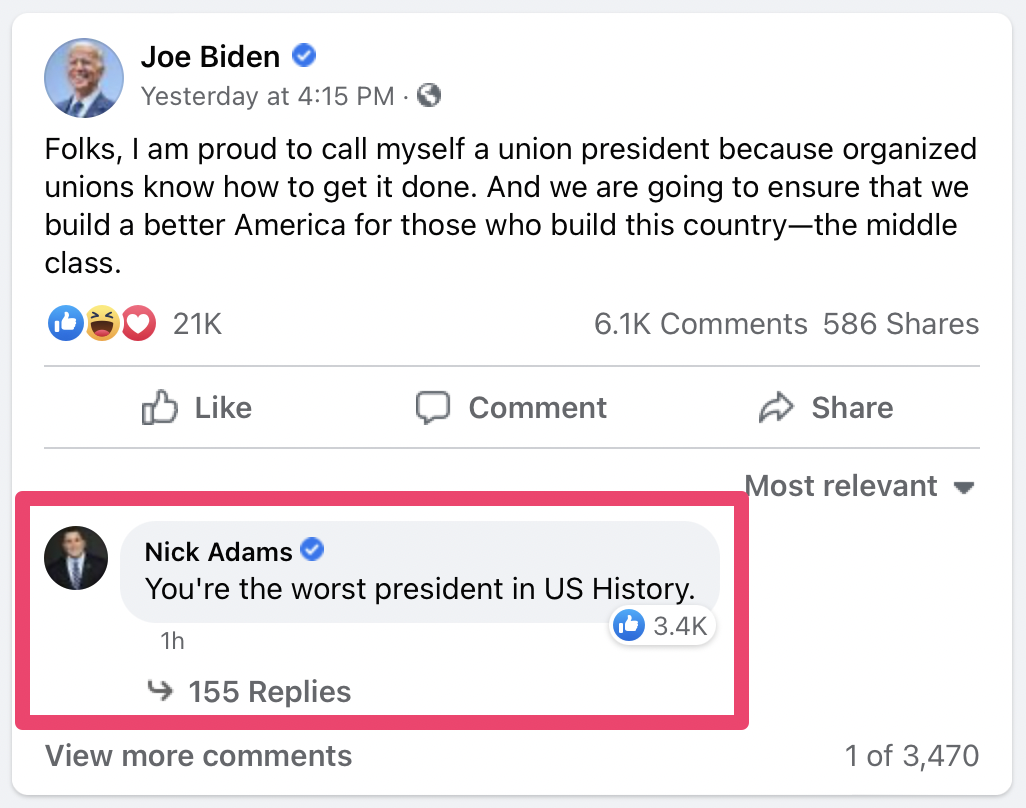
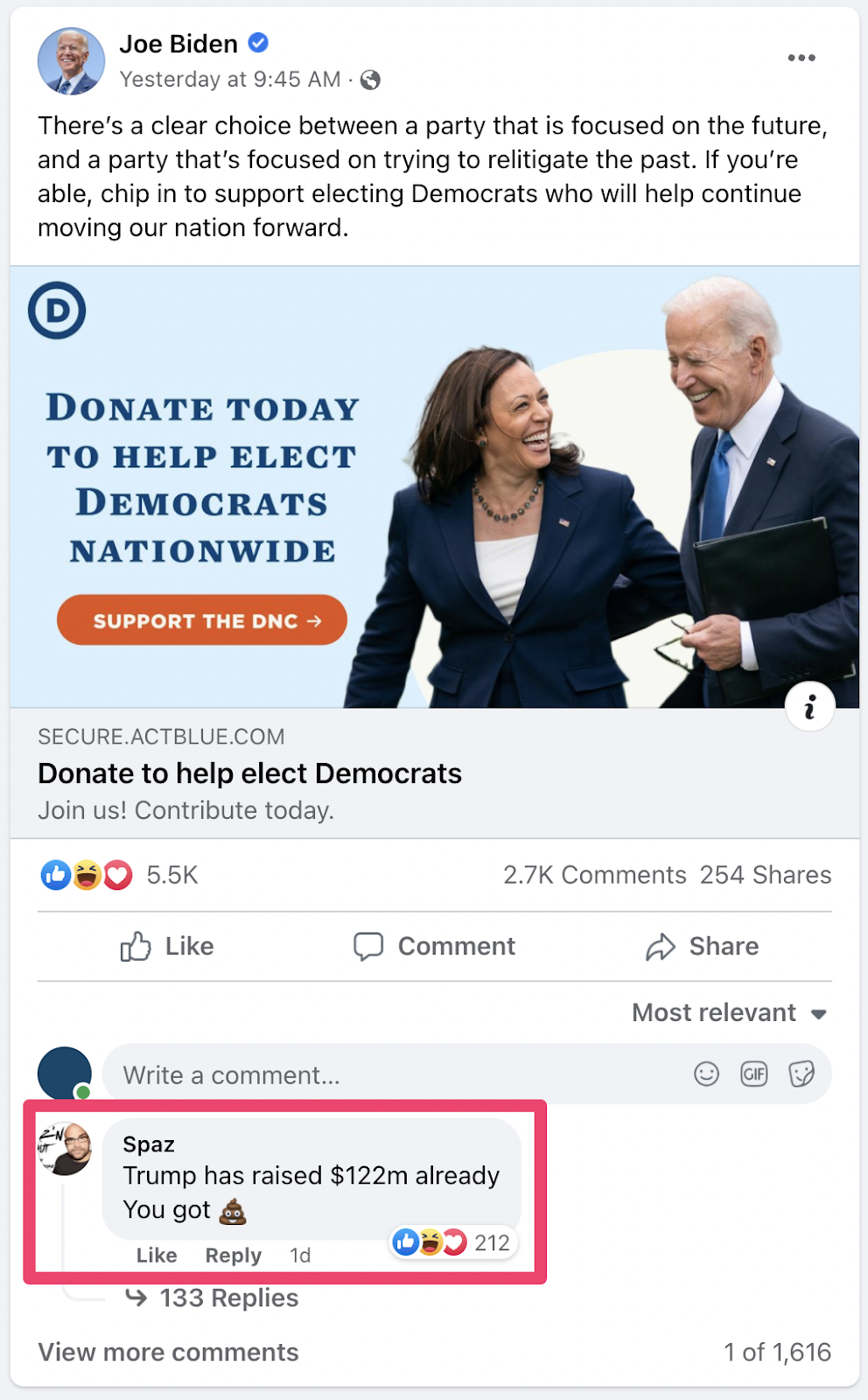
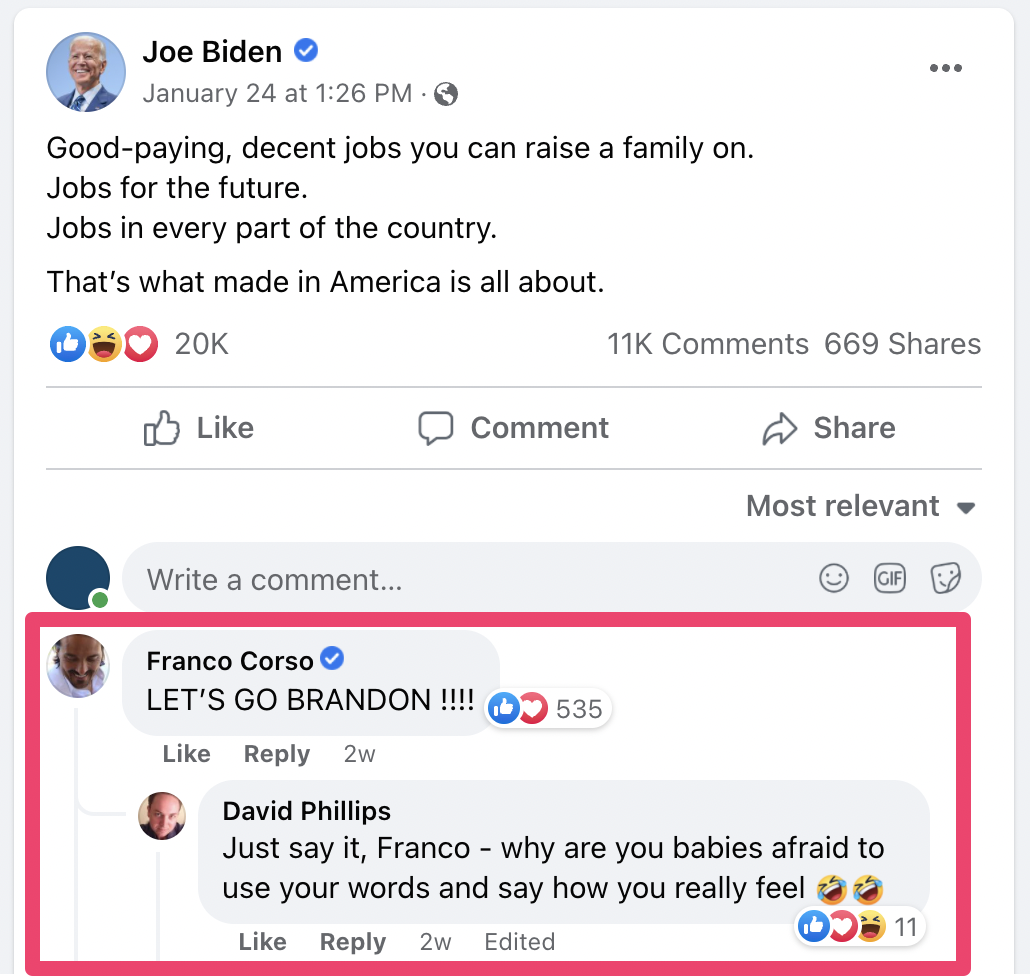
These examples aren’t outliers: data scientists would regularly find that experiments with the largest MSI impact also boosted low-quality content the most. Rather than strengthening quality connections with your friends and family, News Feed often hurt them instead.
And this wasn’t just a Facebook problem — I worked at YouTube and Twitter too, and these issues were prevalent across the board.
A Search Analogy
If engagement-based metrics are the problem, what’s the solution?
There’s a deep analogy with search engines. Just as commenting on a post doesn’t mean you enjoy it — maybe it made you so angry you want to viciously insult the poster — clicks on search pages aren’t necessarily a sign of good results either.
For example, when you search for when was barack obama born?, ideally the answer is displayed at the top of the search result page itself. Clicking is a sign of failure, and there’s no guarantee that the page you click on contains the answer anyways.
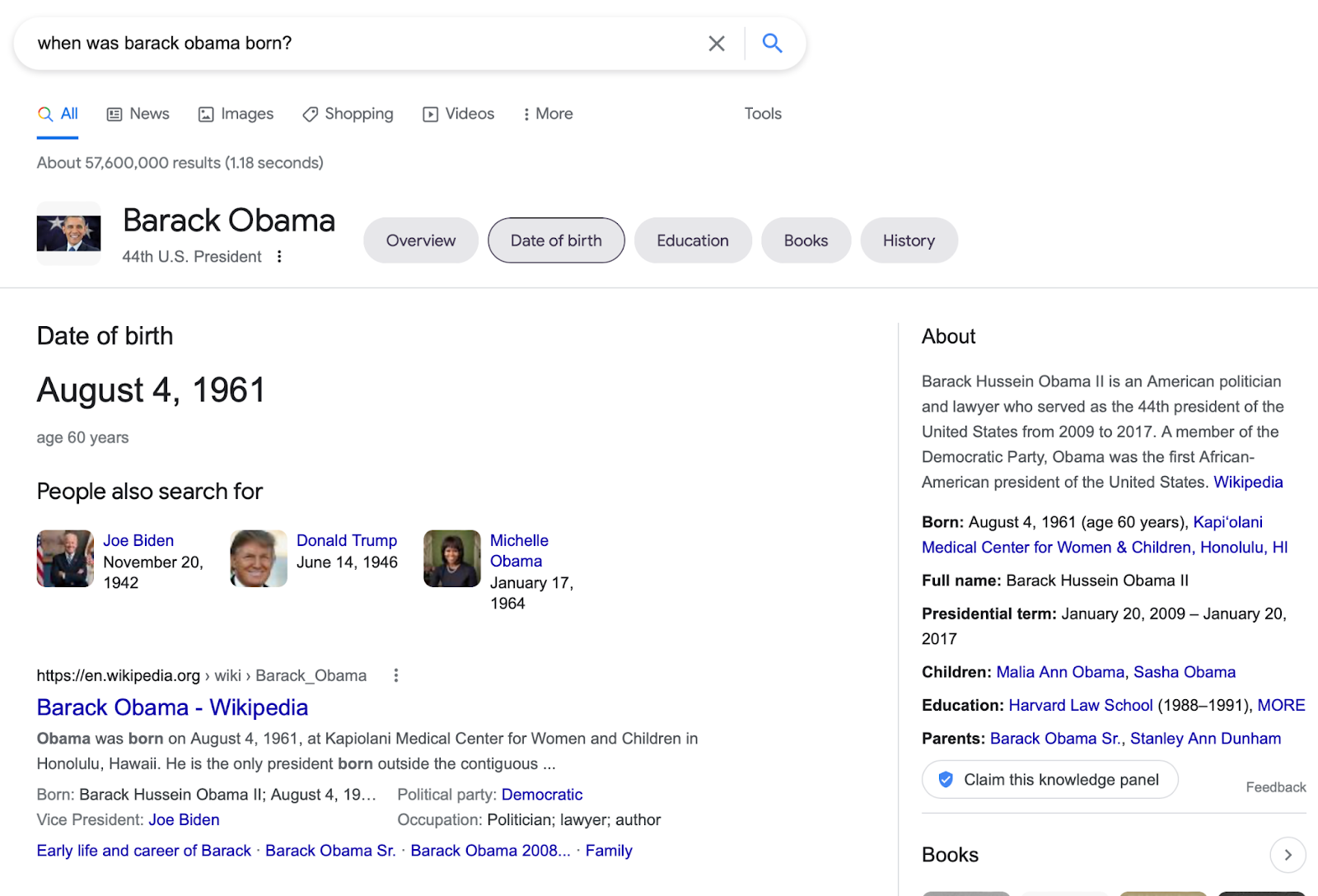
A search engine that optimizes for clicks and engagement would be a poor search engine indeed. And that’s why many search engines like Google and Bing use human evaluation instead. In short, they ask trained human raters to rate the relevance of <search query, search result> pairs; instead of optimizing for clicks, this “human evaluation”-based relevance score is one of their core metrics instead.
For instance, here’s an example of this approach that compares Google and Bing:
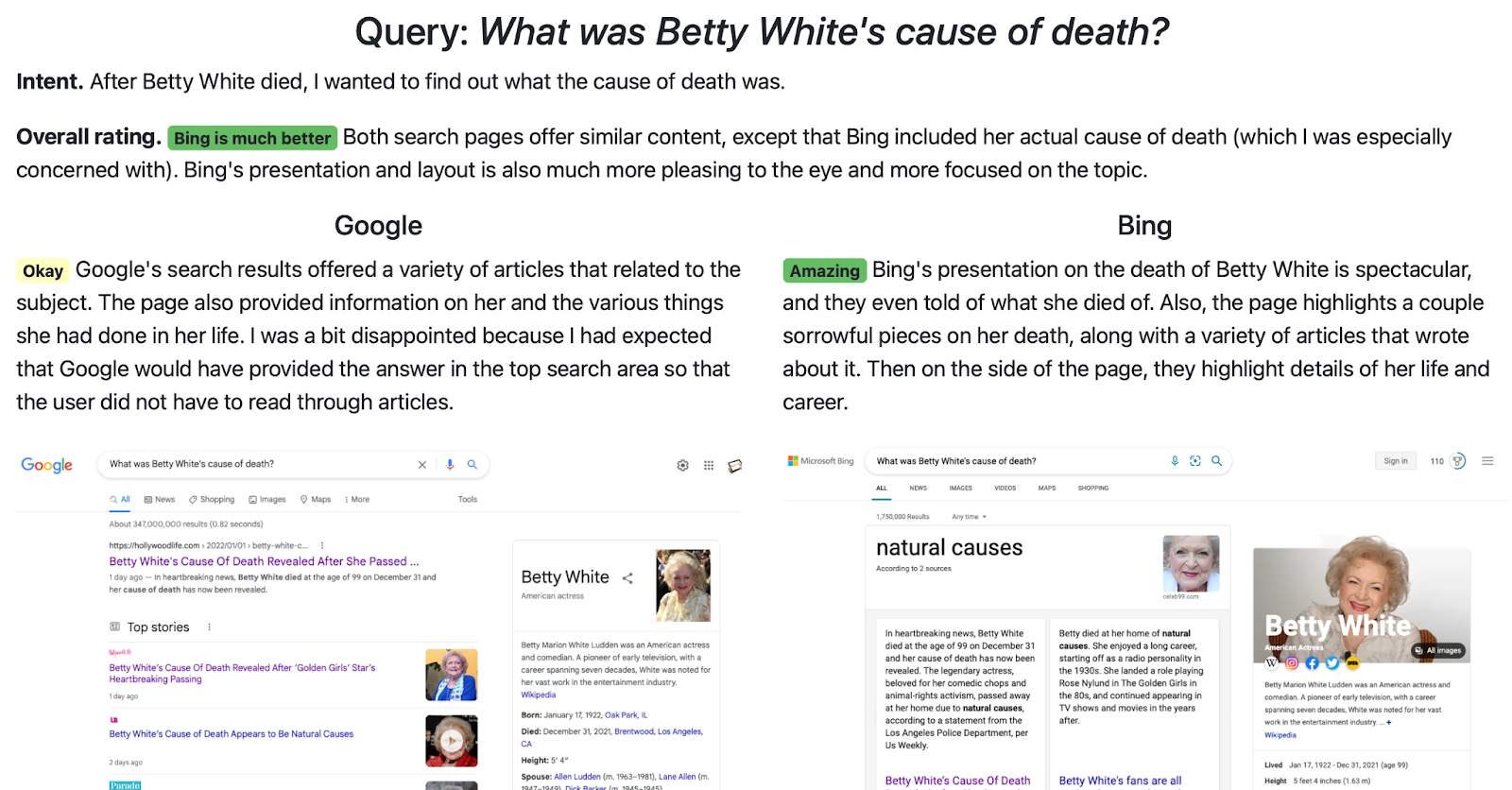
In other words, Google knows that clicks aren’t a good signal, so it asks human raters to score exactly what it cares about: search relevance.
In the same way, MSI and engagement may seem like a good proxy for human values — for healthy, meaningful interactions from friends and family we truly care about — but they’re not. So could Facebook ask human raters to score the exact principle it cares about too?
Human-Based Evaluation
Let's show how this works. Suppose your social media company has one core principle in its mission:
Helping users feel closer to their friends and family.
For example, if Angela’s favorite roommate from college lands her dream job, Angela wants to know. When she can’t make her sister’s wedding due to travel restrictions, she hopes her sister takes a wealth of pictures and videos so she can follow along.
You can encode this product principle into a metric as follows:
- You show Angela a post.
- You ask her how much closer it makes her feel to her friends and family, on a 1-5 point scale.
Importantly, this metric directly matches the principles we laid out!
So imagine every time Facebook has to decide between launching Algorithm A vs. Algorithm B, it shows 1,000 human raters the top 5 posts under each algorithm, and asks them the following:
Did this post make you feel closer to your friends and family on a 1-5 scale?
- 5 - Amazing post. This post reminds me of why I’m close to them and connected to them in the first place. I want to call them on the phone after this, message them to hang out, and more. It’s similar to the feeling I get when I have a fun evening hanging out that I want to capture in my memories.
- 4 - Pretty good post. I enjoyed this post and it reminds me of why I like them, but it doesn't make me feel all that much closer. It’s similar to the feeling of a quick call or a quick lunch that I might enjoy, but forget about a week later.
- 3 - Neutral. It’s a post that I’d just ignore.
- 2 - Pretty bad post. I dislike this post and it makes me a little annoyed or think less of my friends and family. If they called me or messaged me right now, I might just let it go to voicemail. It’s similar to the feeling I get when a family member doesn’t pick up their trash.
- 1 - Horrible post. I hate this post, and it makes me hate them too. I want to unfriend them after this. If I were invited to Thanksgiving with them right now, I’d refuse.
Afterwards, these ratings can be converted into an overall score for Algorithm A and Algorithm B, and the one that wins can be launched.
(As an aside, one question is whether to let your users be your raters, or to use trained experts. I’ve often preferred using expert raters, for a few reasons:
- Users are busy, so don’t respond to your surveys.
- Because these surveys are sent into production, it can be slow to iterate on them — you have to get your flows and wording code reviewed, and wait for deploys.
- You may have very specific definitions of your product principles (for example, does a pet count as “family”? Is pornographic content okay?) encoded in 50 pages of guidelines, but users can’t be expected to perform this level of research.
- Only certain types of people like to respond, so you have difficult problems of bias. I’ve often worked on recommendation systems where we intentionally degraded our algorithms, and saw user survey satisfaction go up!
The potential downside of expert raters is that they may not be representative of your user population, but they can often be calibrated to match. Think of this as similar to a bias-variance tradeoff: surveying your users is in theory unbiased, but there’s large variance in who will respond and how; paid raters, in contrast, exhibit statistical bias because they may not fully match your population of interest, but their training may significantly lower overall variance to an extent that’s worth it.)
Evaluating News Feed
What would this look like? As an example, we asked 100 Surge AI raters to evaluate their News Feeds.
Amazing Bonds
Here were a few examples of amazing posts that raters felt brought them closer to their friends and family.
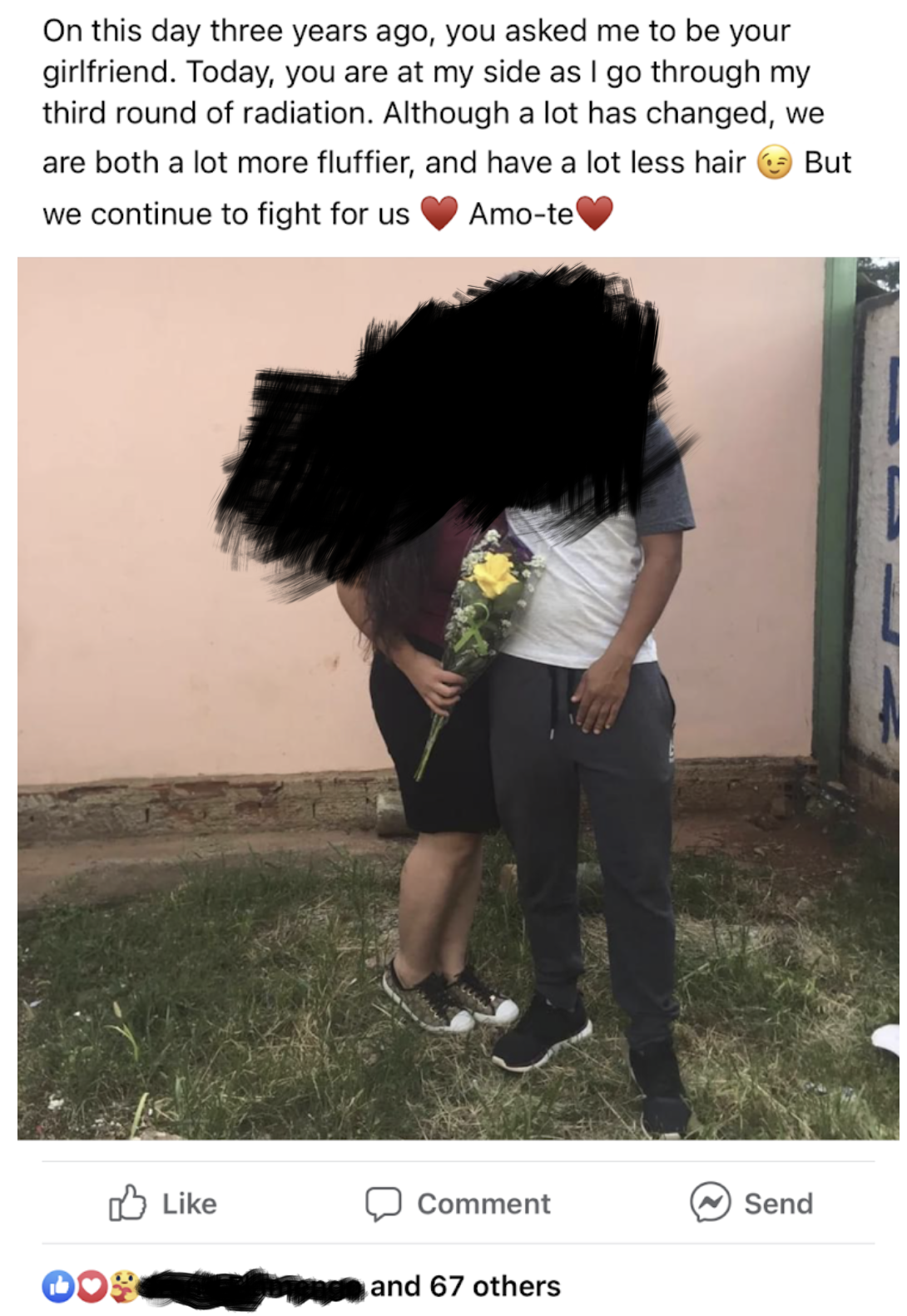
Rating: Amazing. I know both people in this photo, one since childhood. She was recently diagnosed with breast cancer and is now undergoing chemo and radiation. Although she is going through a tough time, this post made me happy because it reminded me how strong she is and made me grateful that she has a wonderful man supporting her through this time.

Rating: Amazing. Seeing this post was a pleasant surprise on my feed. This guy is adorable and it cheers me up to see my family friend with a new member to the crew. I love being updated with the different array of pets that they have, and it makes me happy to think about them playing around with this new puppy. I can’t wait to see them next, and the puppy has such pretty eyes and beautiful coloring on its coat, too!
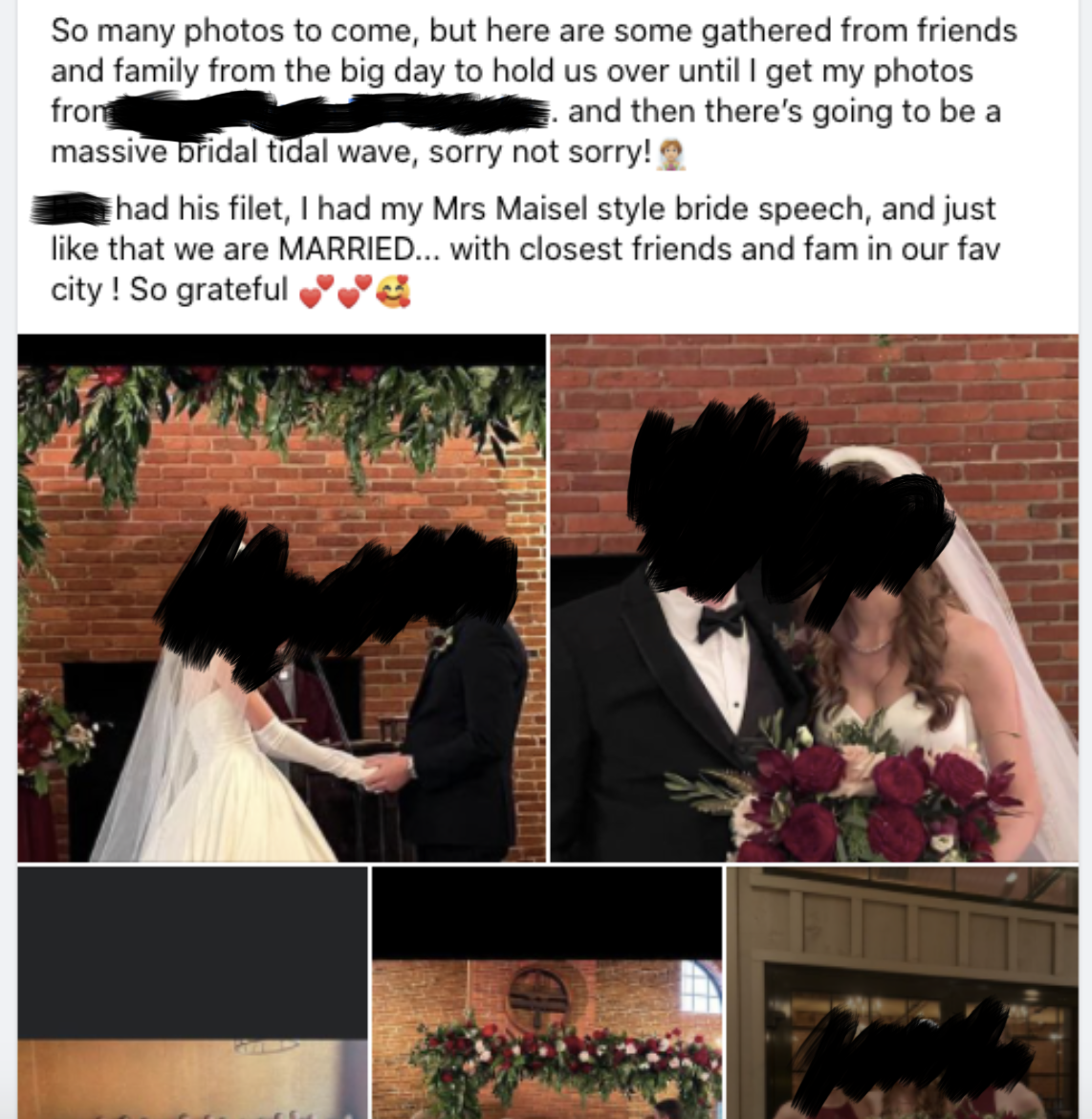
Rating: Amazing. I loved this post. It made me so happy to see a friend of mine so happy. It was a very joyous event and the post made me feel close to her. It also made me feel proud that I had the opportunity to spend such an important milestone with her, and they look beautiful together. I hope she shares these again on her anniversary so we can reminisce!
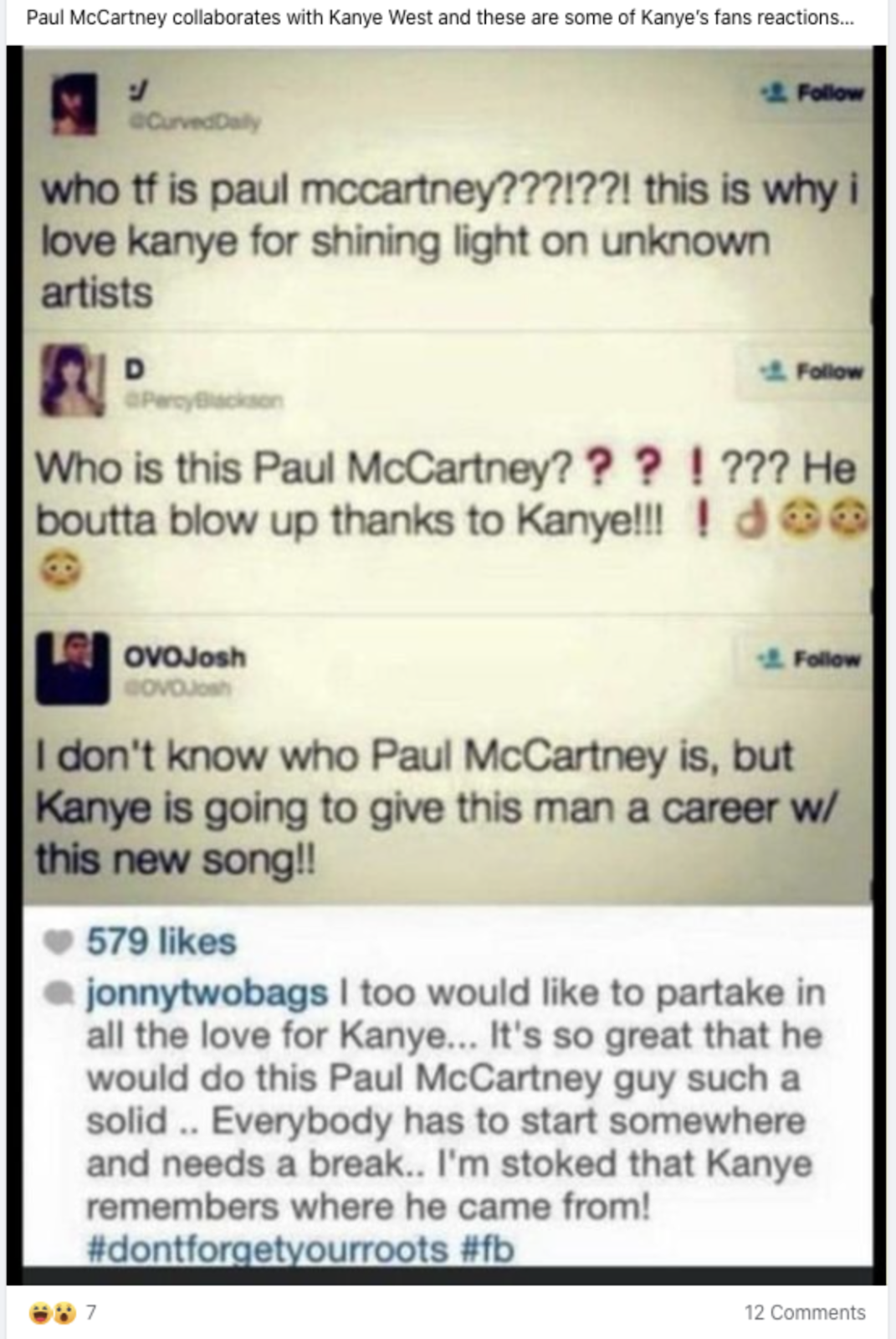
Rating: Amazing. I LOVE this post because it made me laugh out loud and reflect on the truth in it. When you are younger, you look at your parent's references of who they like and scratch your head in wonder. That seems to be the essence of this. But what makes it so great is that it brings out pure joy and laughter. And because of social media and Kanye, this person will learn about Paul McCartney's music and legacy. I haven’t seen my friend in a while because of COVID, but he’s hilarious and always shares the funniest things, so this reminded me of all our fun times together!
Weakening Ties
Here were examples of horrible posts that raters thought weakened their ties.
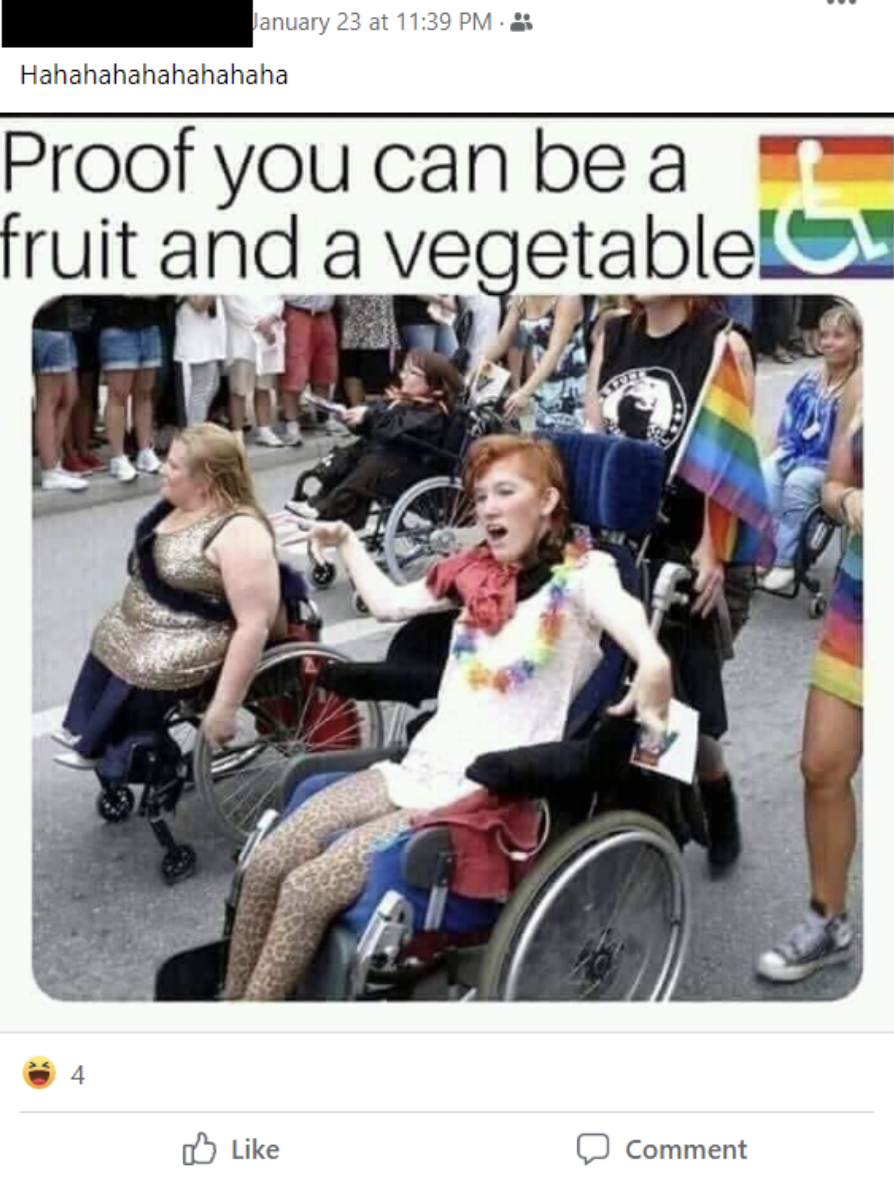
Rating: Horrible. This post pushed me closer to deleting this IRL friend who I've known since 2008. It's one thing to have a dark sense of humor, which he does, but this took it too far. I had a stroke six years ago and am in a wheelchair. I personally was described as possibly remaining "a vegetable" by the doctor who took care of me. Also, my son is trans. Just bad all around.
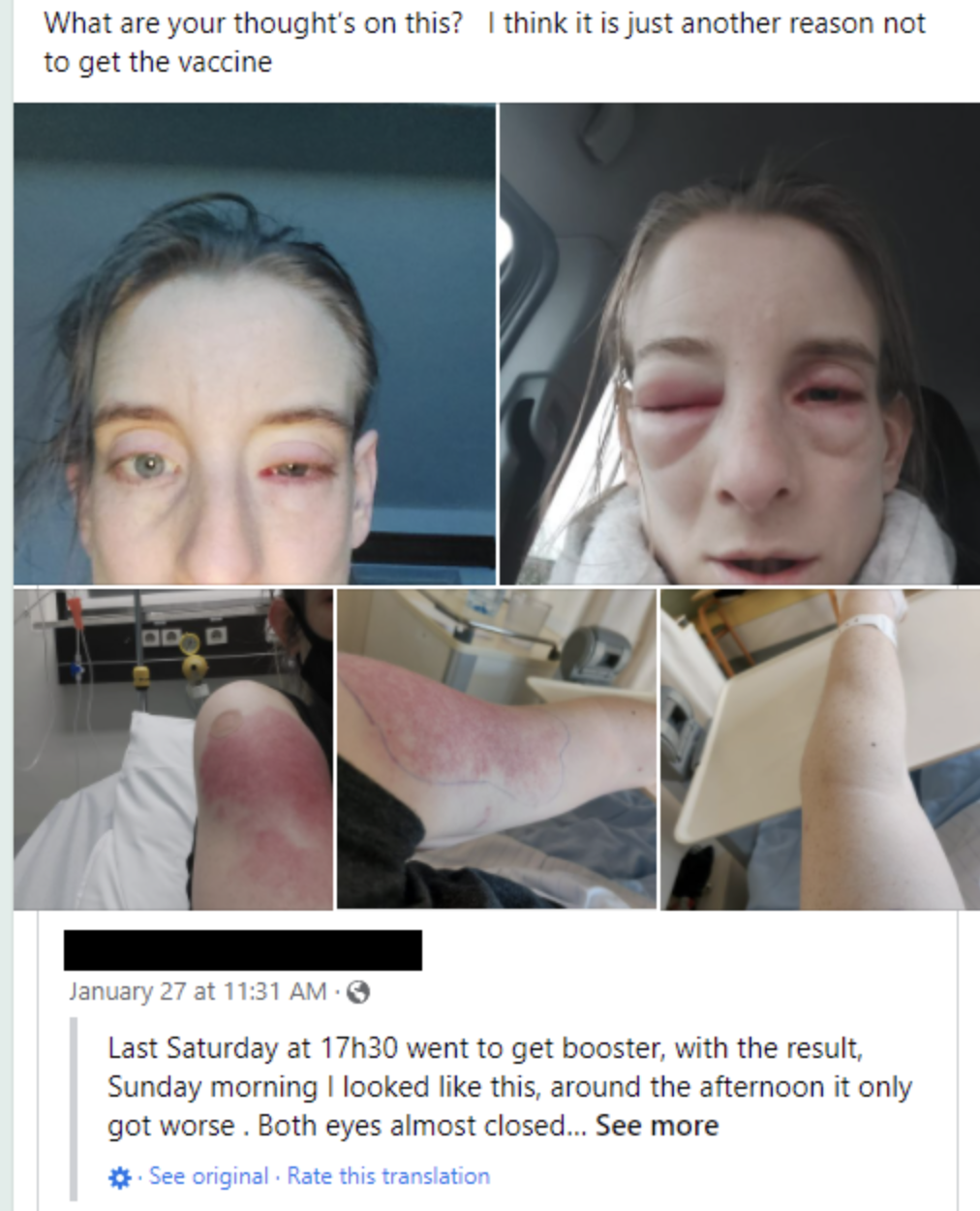
Rating: Horrible. This post is from someone I know that is anti-vaccine. She is constantly sharing and posting misinformative things claiming that the COVID vaccine is harmful and pressuring people not to get it. It makes me so angry that she is trying to push her views and lies on other people in a way that can harm them and leave them at risk of being seriously harmed by the virus if they don't get the vaccine. The photos are just another appeal to emotion to try to scare people. I don't want to unfollow her because we were good friends before the pandemic and the lockdowns, but it's making me feel much less close to her that she's doing this. It makes me want to stop being friends with someone like her.
Applications of Human Eval Scores
What could we do with datasets of thousands of such examples?
OKRs and Dashboards. In the same way that product teams measure daily active users, time spent, and engagement, we can run these human evaluations every day and use them to form a score that’s tracked over time. The overall goal of the company could be to increase the human eval score by 15% in a particular quarter – a concrete way of measuring its progress towards connecting users in ways they actually care about.
For example, in the evaluation we ran, if we consider Horrible posts to have a score of -2 and Amazing posts to have a score of +2, Facebook scored 0.47 on this [-2, +2] scale.
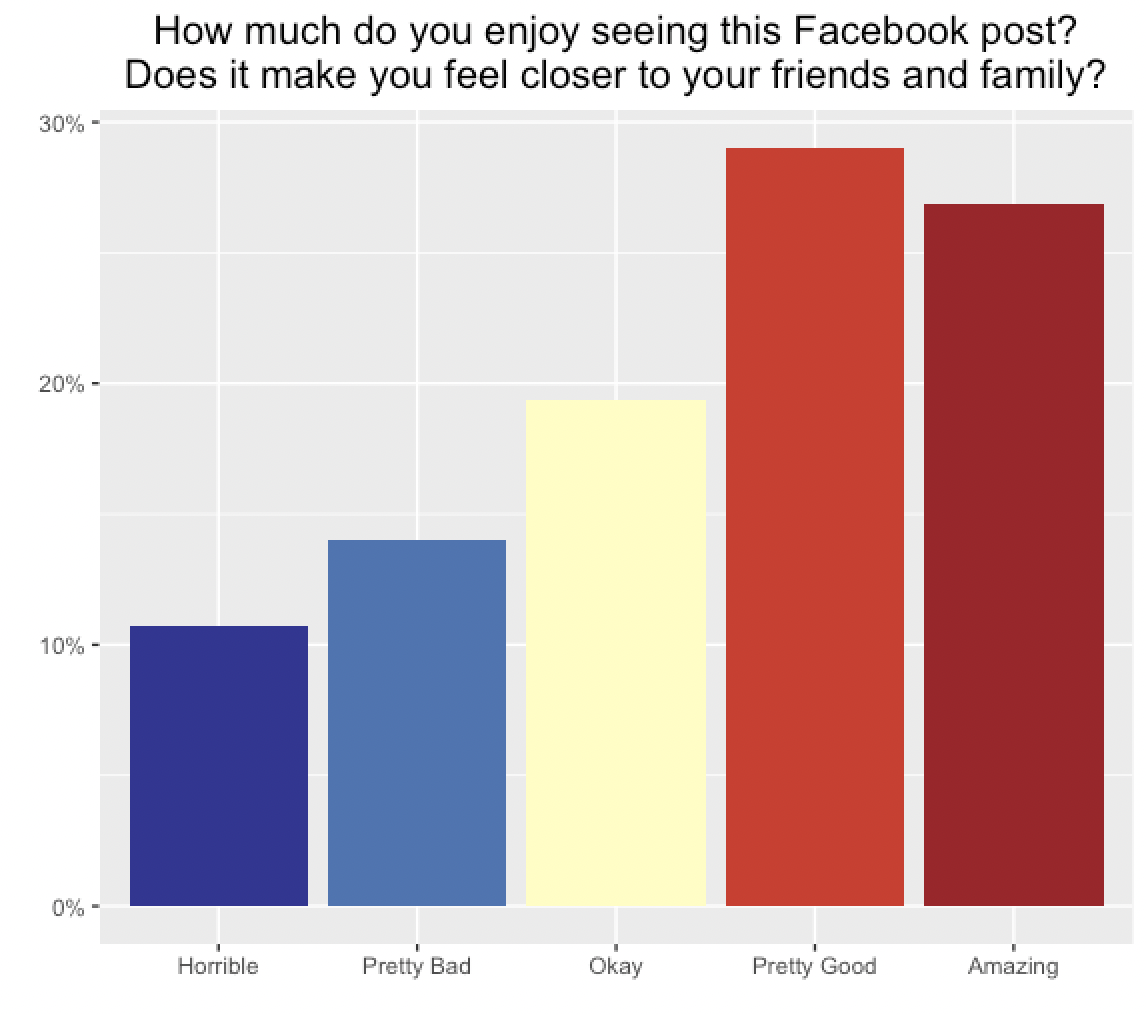
Experiment Launch Decisions. If you’re trying to decide whether to launch News Feed Algorithm A vs. News Feed Algorithm B, you could run an offline side-by-side comparing the two: show raters what their News Feed would look like under A vs. B, and ask them which version makes them feel closer to their friends and family.
In the same way that, traditionally, Facebook might prefer the algorithm with a statistically significant increase in engagement and time spent, now Facebook could launch the algorithm with the higher human eval score.
ML Training. Instead of training News Feed models to rank posts by predicted engagement, ML teams could train algorithms to optimize these human eval scores instead.
Discover New Principles. And, of course, if the friends and family principle seems simplistic — at the end of the day, Facebook cares about many things — other principles can be added to the evaluations too. Picture a Facebook homepage, for example, that always started out with four kinds of posts:
- One that connects you to your friends and family
- One that makes you smile
- One that deepens your interest in a favorite hobby
- One that teaches you something new about the world
Would Facebook be more loved today, if it had injected human values into its metrics and goals instead?
Summary
In short: it 's possible to measure Facebook’s ability to bring friends and family closer together! And to do so in a fast, rigorous, and scalable way. This means we can take all the advantages of data-driven development — running A/B tests, setting OKRs, forming hypotheses based on metrics and data — without sacrificing core product principles.
Finally, Facebook is just one of many platforms struggling with the unintended consequences of engagement metrics. Can this human evaluation method be extended to other platforms too? Yes — we’ll cover Twitter and YouTube in our next post! Until then, let's all sing along to John Lennon's classic:
Imagine there’s no like button
It’s easy if you try
No hell below us
No metrics to satisfy
Imagine no engagement
I wonder if you can
No need for clicks or likes
A brotherhood of man
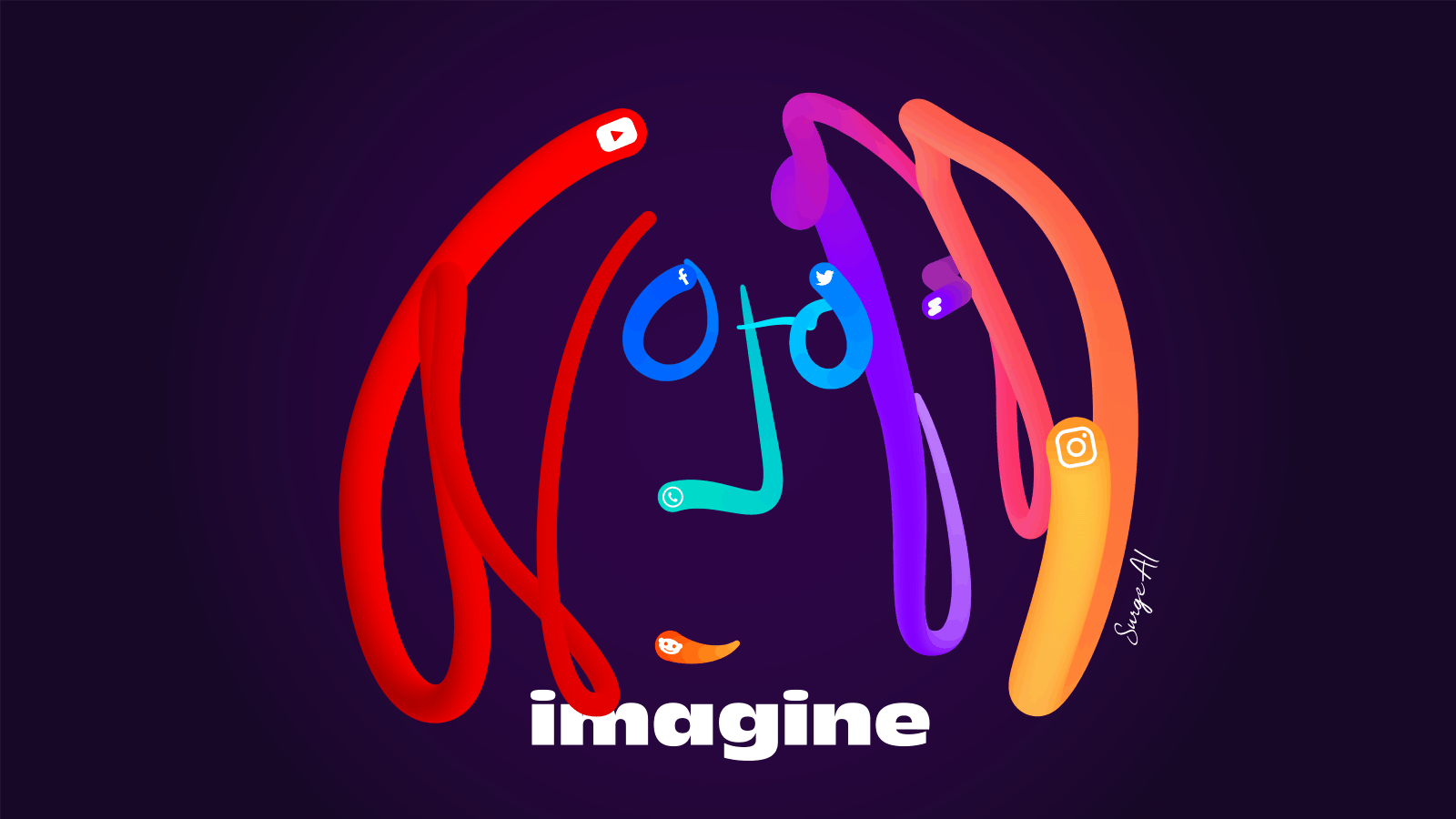
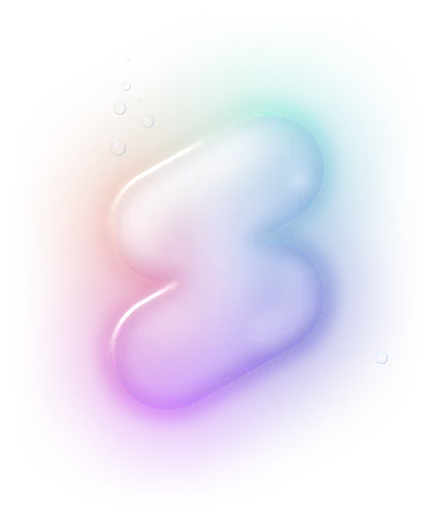
Data Labeling 2.0 for Rich, Creative AI
Superintelligent AI, meet your human teachers. Our data labeling platform is designed from the ground up to train the next generation of AI — whether it’s systems that can code in Python, summarize poetry, or detect the subtleties of toxic speech. Use our powerful data labeling workforce and tools to build the rich, human-powered datasets you need today.